Deep learning network based on group convolution feature topological space and training method thereof
A deep learning network and convolution technology, which is applied to the deep learning network and its training field based on group convolution feature topological space, can solve the problems of lack of global spatial features, slow training speed, and a large number of samples, so as to speed up network training. and convergence speed, the effect of accelerating training convergence speed
- Summary
- Abstract
- Description
- Claims
- Application Information
AI Technical Summary
Problems solved by technology
Method used
Image
Examples
Embodiment Construction
[0047] In order to better understand the technical content of the present invention, specific embodiments are given together with the attached drawings for description as follows.
[0048] Such as figure 1 As shown, the present invention provides a detailed network structure diagram, including: convolution feature extraction layer L1, group convolution topology layer L2 and deep feature recognition layer L3.
[0049] The convolutional feature extraction layer L1 uses a traditional CNN network to extract multi-channel convolutional features, including a first convolutional layer Conv1, a first pooling layer Pool1, a second convolutional layer Conv2, and a second pooling layer Pool2.
[0050] The group convolution topology layer L2 includes group convolution feature layer Group Features, graph network input layer GraphInput, graph neural network hidden layer Hidden Layer, and graph network output layer Graph Output, which is responsible for extracting CNN features under differen...
PUM
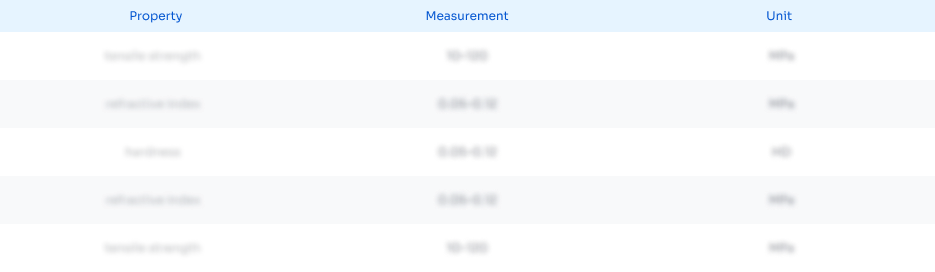
Abstract
Description
Claims
Application Information
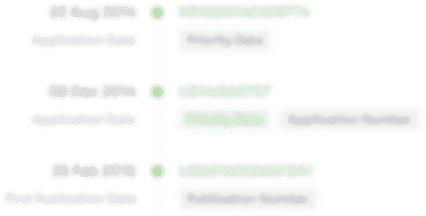
- R&D
- Intellectual Property
- Life Sciences
- Materials
- Tech Scout
- Unparalleled Data Quality
- Higher Quality Content
- 60% Fewer Hallucinations
Browse by: Latest US Patents, China's latest patents, Technical Efficacy Thesaurus, Application Domain, Technology Topic, Popular Technical Reports.
© 2025 PatSnap. All rights reserved.Legal|Privacy policy|Modern Slavery Act Transparency Statement|Sitemap|About US| Contact US: help@patsnap.com