Image semantic segmentation method based on PU-Learning
A semantic segmentation and image technology, applied in the field of computer vision, can solve the problems of difficult to guarantee the quality of semantic segmentation, high cost, weak supervision, etc., to achieve good semantic segmentation quality and improve the effect of labeling speed.
- Summary
- Abstract
- Description
- Claims
- Application Information
AI Technical Summary
Problems solved by technology
Method used
Image
Examples
Embodiment Construction
[0019] The following will clearly and completely describe the technical solutions in the embodiments of the present invention with reference to the accompanying drawings in the embodiments of the present invention. Obviously, the described embodiments are only some, not all, embodiments of the present invention. Based on the embodiments of the present invention, all other embodiments obtained by persons of ordinary skill in the art without making creative efforts belong to the protection scope of the present invention.
[0020] see figure 1 , the present invention provides a technical solution: a method for image semantic segmentation based on PU-Learning, comprising the following steps:
[0021] S1. Data preparation, and in the image database to be trained, there is at least one pixel-level image label for each category (it is necessary to uniformly set the label of unlabeled samples to 0, and label the labels of other samples of different categories from 1 );
[0022] S2. ...
PUM
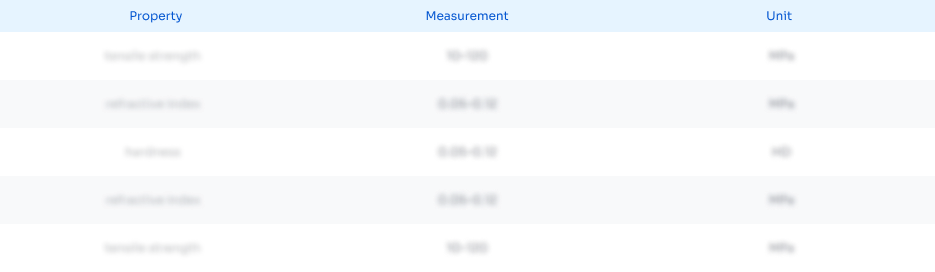
Abstract
Description
Claims
Application Information
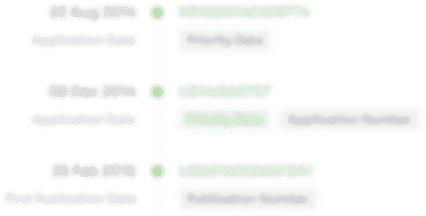
- R&D Engineer
- R&D Manager
- IP Professional
- Industry Leading Data Capabilities
- Powerful AI technology
- Patent DNA Extraction
Browse by: Latest US Patents, China's latest patents, Technical Efficacy Thesaurus, Application Domain, Technology Topic, Popular Technical Reports.
© 2024 PatSnap. All rights reserved.Legal|Privacy policy|Modern Slavery Act Transparency Statement|Sitemap|About US| Contact US: help@patsnap.com