Graph-based convolutional network training method, device and system
A convolutional network and training method technology, applied in the field of machine learning, can solve the problems of restricting the application of GCN model, increasing the time of GCN model training, and unacceptable model training time, so as to reduce the amount of calculation and training time, and avoid the amount of calculation huge effect
- Summary
- Abstract
- Description
- Claims
- Application Information
AI Technical Summary
Problems solved by technology
Method used
Image
Examples
Embodiment Construction
[0055] Exemplary embodiments of the present disclosure will be described in more detail below with reference to the accompanying drawings. Although exemplary embodiments of the present disclosure are shown in the drawings, it should be understood that the present disclosure may be embodied in various forms and should not be limited by the embodiments set forth herein. Rather, these embodiments are provided for more thorough understanding of the present disclosure and to fully convey the scope of the present disclosure to those skilled in the art.
[0056] Graph learning is meaningful for business scenarios. Therefore, when the relationship between the entities corresponding to the nodes and the entities corresponding to the edges in the graph is determined based on the business scenario, the graph is endowed with business and technical meanings. According to the business scenario The technical problems and business problems to be solved perform the corresponding graph learning...
PUM
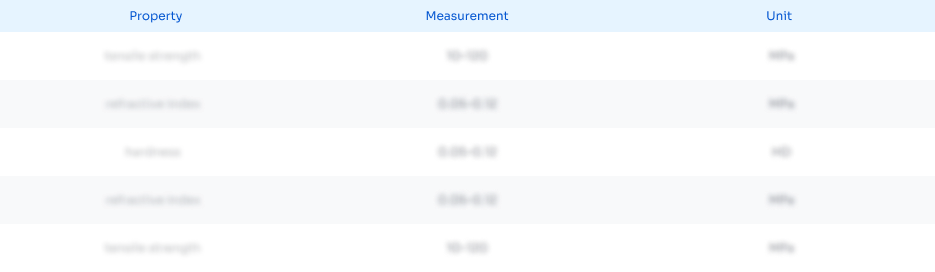
Abstract
Description
Claims
Application Information
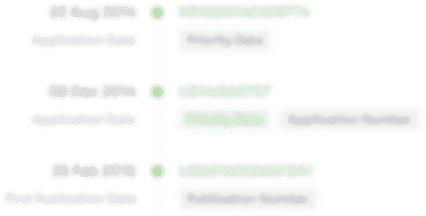
- R&D
- Intellectual Property
- Life Sciences
- Materials
- Tech Scout
- Unparalleled Data Quality
- Higher Quality Content
- 60% Fewer Hallucinations
Browse by: Latest US Patents, China's latest patents, Technical Efficacy Thesaurus, Application Domain, Technology Topic, Popular Technical Reports.
© 2025 PatSnap. All rights reserved.Legal|Privacy policy|Modern Slavery Act Transparency Statement|Sitemap|About US| Contact US: help@patsnap.com