High-speed Traffic Flow Prediction Method Based on Multimodal Fusion and Graph Attention Mechanism
A prediction method and attention technology, applied in the field of traffic information, can solve the problems of gradient disappearance, limited ability to capture complex topology features, and error accumulation, and achieve the effect of improving prediction accuracy.
- Summary
- Abstract
- Description
- Claims
- Application Information
AI Technical Summary
Problems solved by technology
Method used
Image
Examples
Embodiment Construction
[0044] In order to describe the present invention more specifically, the technical solutions of the present invention will be described in detail below in conjunction with the accompanying drawings and specific embodiments.
[0045] The high-speed traffic flow prediction method based on multimodal fusion and graph attention mechanism of the present invention includes the following steps:
[0046] (1) Feature data collection and preprocessing.
[0047] 1.1 Calculation of vehicle speed and road speed
[0048] For a motor vehicle driving on the expressway, the camera on the expressway should capture the vehicle at continuous checkpoints, and obtain discrete vehicle position data at different time points (p 0 ,p 1 ,p 2 ,···,p t ), so that the average velocity (v 0 ,v 1 ,v 2 ,···,v t-1 ),in However, affected by uncontrollable factors such as equipment and weather conditions in real situations, the recognition of license plates is not always correct, which may cause data d...
PUM
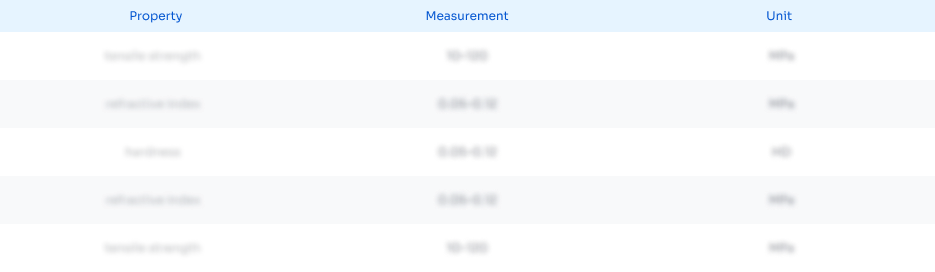
Abstract
Description
Claims
Application Information
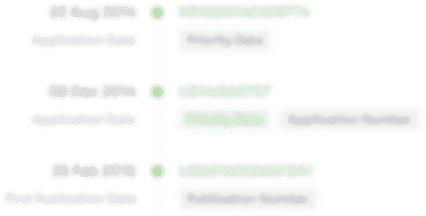
- R&D Engineer
- R&D Manager
- IP Professional
- Industry Leading Data Capabilities
- Powerful AI technology
- Patent DNA Extraction
Browse by: Latest US Patents, China's latest patents, Technical Efficacy Thesaurus, Application Domain, Technology Topic.
© 2024 PatSnap. All rights reserved.Legal|Privacy policy|Modern Slavery Act Transparency Statement|Sitemap