Image classification network training method and device, equipment and storage medium
A classification network and training method technology, applied in the field of image recognition, can solve the problems of complex implementation, high cost, and affecting the training efficiency and classification accuracy of the image classification network
- Summary
- Abstract
- Description
- Claims
- Application Information
AI Technical Summary
Problems solved by technology
Method used
Image
Examples
Embodiment 1
[0035] figure 1 It is a flow chart of an image classification network training method provided by Embodiment 1 of the present invention. This embodiment is applicable to the situation where part of the data is selected from a large amount of data to be labeled to train the image classification network. Specifically, the image classification network training method can be executed by an image classification network training device, and the image classification network training device can be realized by software and / or hardware, and integrated in the device. Wherein, the equipment includes but is not limited to: desktop computer, notebook computer, cloud server, etc. The specific content of the labeled sample set or unlabeled data is not limited here. For example, in the process of identifying and classifying lung images in the field of medical imaging, the labeled and labeled image data can be used as The labeled sample set, and the unlabeled data refer to unlabeled lung imag...
Embodiment 2
[0057] image 3 It is a flowchart of a training method for an image classification network provided by Embodiment 2 of the present invention. This embodiment optimizes the process of selecting target data, updating the image classification network, and labeling results on the basis of the above-mentioned embodiments. The processing is described in detail. It should be noted that for technical details not exhaustively described in this embodiment, reference may be made to any of the foregoing embodiments.
[0058] In this embodiment, selecting target data from unlabeled data based on the policy network and evaluation network to label, specifically includes: based on the action vector a of the policy network, the unlabeled data is selected from large to small according to the selected probability value Sorting is performed, and a set amount of data with the highest probability value is selected as the target data for labeling; wherein, the action vector a is determined accordin...
Embodiment 3
[0083] Figure 5 It is a flow chart of an image classification network training method provided by Embodiment 3 of the present invention. This embodiment is optimized on the basis of the above embodiments, and specifically describes the update process of the policy network and the evaluation network. It should be noted that for technical details not exhaustively described in this embodiment, reference may be made to any of the foregoing embodiments.
[0084] In this embodiment, updating the policy network and the evaluation network according to the buffered data includes: calculating a reward function corresponding to the policy network and the evaluation network according to the buffered data, and the reward function is a Q-value function; calculating according to the buffered data The loss function corresponding to the policy network and the evaluation network; with the optimization goal of maximizing the reward function and minimizing the loss function, adjust the network p...
PUM
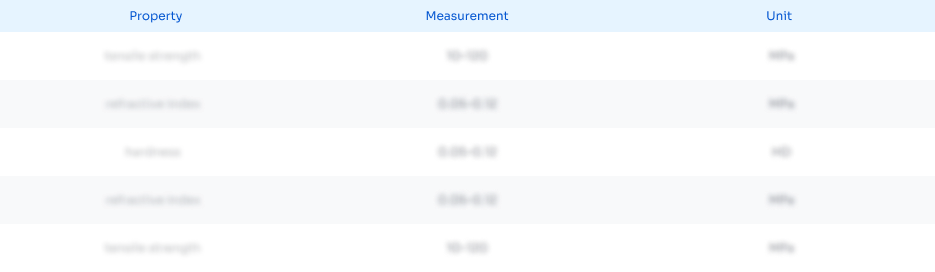
Abstract
Description
Claims
Application Information
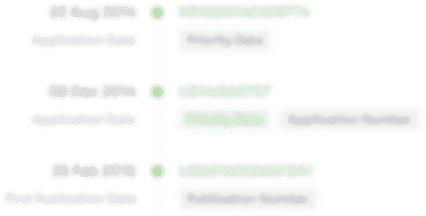
- R&D
- Intellectual Property
- Life Sciences
- Materials
- Tech Scout
- Unparalleled Data Quality
- Higher Quality Content
- 60% Fewer Hallucinations
Browse by: Latest US Patents, China's latest patents, Technical Efficacy Thesaurus, Application Domain, Technology Topic, Popular Technical Reports.
© 2025 PatSnap. All rights reserved.Legal|Privacy policy|Modern Slavery Act Transparency Statement|Sitemap|About US| Contact US: help@patsnap.com