Deep learning model training method for fine portrait picture segmentation
A technology of deep learning and image segmentation, applied in neural learning methods, image analysis, biological neural network models, etc., can solve problems such as time-consuming, cost cannot be realized on a large scale, and edge accuracy cannot be met, and achieve cost-saving effects
- Summary
- Abstract
- Description
- Claims
- Application Information
AI Technical Summary
Problems solved by technology
Method used
Image
Examples
Embodiment Construction
[0052] The present invention will be described in further detail below in conjunction with the accompanying drawings and specific embodiments.
[0053] The embodiment process implemented according to the complete method of the content of the present invention is as follows:
[0054] 1) Collect and acquire portrait pictures, with the portrait as the foreground, the foreground area in the portrait picture has been marked as 1, and the background area has been marked as 0, forming a binary portrait / background annotation map, such as figure 1 As shown, constitute the training data set;
[0055] 2) Use the Canny edge algorithm to detect the boundary between the portrait and the background on the binarized portrait / background annotation map;
[0056] 3) Use the adaptive expansion operator to expand the boundary result obtained in step 2) to form the edge area, and obtain the portrait edge / background area annotation map M bound ,Such as figure 2 As shown, in the portrait edge / bac...
PUM
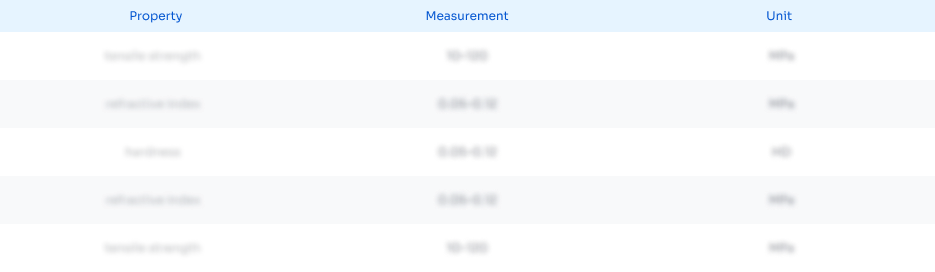
Abstract
Description
Claims
Application Information
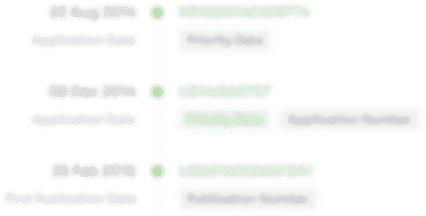
- R&D Engineer
- R&D Manager
- IP Professional
- Industry Leading Data Capabilities
- Powerful AI technology
- Patent DNA Extraction
Browse by: Latest US Patents, China's latest patents, Technical Efficacy Thesaurus, Application Domain, Technology Topic.
© 2024 PatSnap. All rights reserved.Legal|Privacy policy|Modern Slavery Act Transparency Statement|Sitemap