Construction method of wind driven generator bearing fault identification model based on deep learning
A technology for wind turbines and construction methods, applied in neural learning methods, biological neural network models, neural architectures, etc., can solve problems such as low accuracy, high labor costs, and reduced identification accuracy, and reduce downtime or maintenance. cost, reduce labor costs, and improve the effect of accuracy
- Summary
- Abstract
- Description
- Claims
- Application Information
AI Technical Summary
Problems solved by technology
Method used
Image
Examples
Embodiment Construction
[0030] The construction method of the wind turbine bearing fault identification model based on deep learning is realized by the following steps:
[0031] Step 1. Fault type and quantity of preset bearings
[0032] Fault type 1: The state of the bearing at the transmission end is electric corrosion of the inner and outer rings, and the state of the bearing at the non-drive end is normal;
[0033] Fault type 2: The state of the bearing at the drive end is normal, and the state of the bearing at the non-drive end is electric corrosion of the inner and outer rings;
[0034] Fault type three: the state of the bearing at the drive end is normal, and the state of the bearing at the non-drive end is normal;
[0035] Fault type four: The state of the bearing at the transmission end is that the inner and outer rings are peeled off, and the state of the bearing at the non-drive end is normal;
[0036] Fault type 5: The state of the bearing at the drive end is normal, and the state of t...
PUM
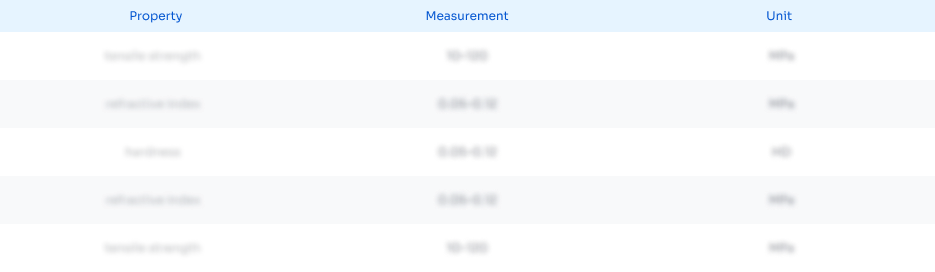
Abstract
Description
Claims
Application Information
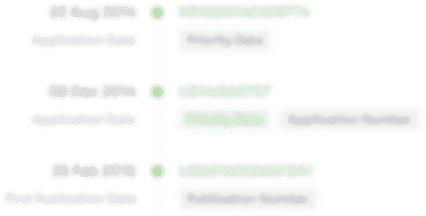
- R&D Engineer
- R&D Manager
- IP Professional
- Industry Leading Data Capabilities
- Powerful AI technology
- Patent DNA Extraction
Browse by: Latest US Patents, China's latest patents, Technical Efficacy Thesaurus, Application Domain, Technology Topic, Popular Technical Reports.
© 2024 PatSnap. All rights reserved.Legal|Privacy policy|Modern Slavery Act Transparency Statement|Sitemap|About US| Contact US: help@patsnap.com