Binding recommendation method and system based on graph convolutional neural network
A convolutional neural network and recommendation method technology, applied in the field of bundled recommendation methods and systems based on graph convolutional neural networks, can solve the problem of inability to balance the weight relationship between users, bundles and items, the decision reference relationship is not comprehensive enough, and the recommendation results are not accurate enough and objective problems, to achieve the effect of good recommendation performance
- Summary
- Abstract
- Description
- Claims
- Application Information
AI Technical Summary
Problems solved by technology
Method used
Image
Examples
Embodiment approach 1
[0109] Implementation mode one: if Figure 10 In the middle branch of , the user wants to use the user's interaction with the item and the bundle history tracked by the platform to recommend new bundles to the user. The platform here can be any e-commerce and content platform, corresponding to any items that can form a bundle, such as commodities, food, places, music, books, movies, news, etc.
[0110] First, the historical interaction between the user and the item, the historical interaction between the user and the bundle, and the composition information of the bundle are formalized into a matrix to obtain the user-bundle historical interaction matrix X M×N , user-item historical interaction matrix Y M×O and bundle-item affiliation matrix Z N×O , a unified heterogeneous graph can be described by three matrices. where node by user node The binding node b∈B and the item node i∈I are composed, and the edge E is composed of the corresponding x ub = 1 user-bundle interact...
Embodiment approach 2
[0113] Implementation mode two: if Figure 10 In the left branch, the user wants to recommend a new bundle to the user by using the interaction between the user and the bundle history tracked by the platform. The platform here can be any e-commerce and content platform, corresponding to any items that can form a bundle, such as commodities, food, places, music, books, movies, news, etc.
[0114] First, the historical interaction between the user and the bundle, and the composition information of the bundle are formalized into a matrix, and the user-bundle history interaction matrix X is obtained M×N and bundle-item affiliation matrix Z N×O , a unified heterogeneous graph can be described by two matrices. where node by user node The binding node b∈B and the item node i∈I are composed, and the edge E is composed of the corresponding x ub = 1 user-bundle interaction edge (u,b) and corresponding z bi Bundle = 1 - items are composed of dependent edges (b,i). For user and b...
Embodiment approach 3
[0117] Implementation mode three: if Figure 10 In the right branch of , the user wants to use the historical interaction between the user and the item tracked by the platform to recommend new bundles to the user. The platform here can be any e-commerce and content platform, corresponding to any items that can form a bundle, such as commodities, food, places, music, books, movies, news, etc.
[0118] First, the historical interaction between the user and the item and the composition information of the bundle are formalized into a matrix, and the user-item historical interaction matrix Y is obtained M×O and bundle-item affiliation matrix Z N×O , a unified heterogeneous graph can be described by two matrices. where node by user node The binding node b∈B and the item node i∈I are composed, and the edge E is composed of the corresponding y ui = 1 user-item interaction edge (u,i) and corresponding z bi Bundle = 1 - items are composed of dependent edges (b,i). For user and ...
PUM
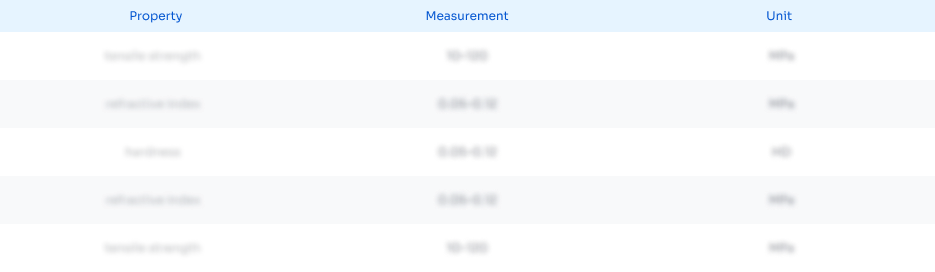
Abstract
Description
Claims
Application Information
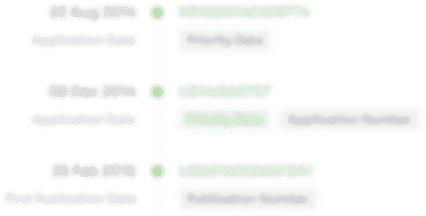
- R&D
- Intellectual Property
- Life Sciences
- Materials
- Tech Scout
- Unparalleled Data Quality
- Higher Quality Content
- 60% Fewer Hallucinations
Browse by: Latest US Patents, China's latest patents, Technical Efficacy Thesaurus, Application Domain, Technology Topic, Popular Technical Reports.
© 2025 PatSnap. All rights reserved.Legal|Privacy policy|Modern Slavery Act Transparency Statement|Sitemap|About US| Contact US: help@patsnap.com