End-to-end network slice resource allocation algorithm based on deep reinforcement learning
A technology for reinforcement learning and resource allocation, applied in the field of communication networks
- Summary
- Abstract
- Description
- Claims
- Application Information
AI Technical Summary
Problems solved by technology
Method used
Image
Examples
Embodiment Construction
[0104]The present invention designs an end-to-end network slicing resource allocation algorithm based on deep reinforcement learning. This method comprehensively considers the access side and the core side, dynamically and reasonably allocates resources, and aims to improve the access rate of the system. In order to obtain the best resource allocation strategy, the factors that affect the access rate in the environment are trained using deep reinforcement learning to obtain a network model. In order to solve the value of the access rate under the determination of wireless resource allocation, an end-to-end resource mapping algorithm is designed for the access side and the core side. With these prerequisites, it is possible to use the trained network to reasonably manage the dynamically changing environment Resource allocation. The specific implementation method is as follows:
[0105] 1. End-to-end model and wireless resource initialization
[0106] A. End-to-end model acces...
PUM
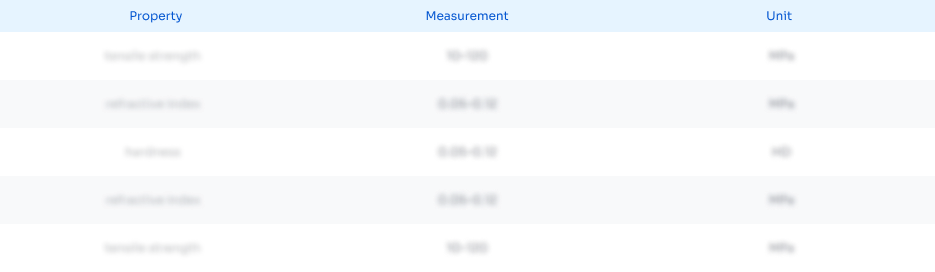
Abstract
Description
Claims
Application Information
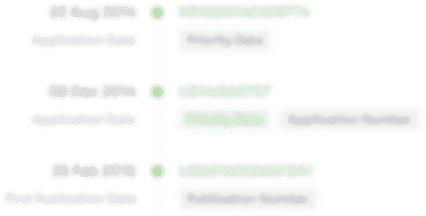
- R&D
- Intellectual Property
- Life Sciences
- Materials
- Tech Scout
- Unparalleled Data Quality
- Higher Quality Content
- 60% Fewer Hallucinations
Browse by: Latest US Patents, China's latest patents, Technical Efficacy Thesaurus, Application Domain, Technology Topic, Popular Technical Reports.
© 2025 PatSnap. All rights reserved.Legal|Privacy policy|Modern Slavery Act Transparency Statement|Sitemap|About US| Contact US: help@patsnap.com