Single image super-resolution reconstruction method guided by self-paradigm learning
A super-resolution reconstruction and low-resolution technology, applied in the field of image super-resolution reconstruction, can solve the problems of low similarity of matching image blocks, loss of image detail information, small search space of image blocks, etc., and achieve high-frequency details Sufficient information, improved objective evaluation indicators, and improved algorithm efficiency
- Summary
- Abstract
- Description
- Claims
- Application Information
AI Technical Summary
Problems solved by technology
Method used
Image
Examples
Embodiment Construction
[0092] The following is a further description of the technical solution of the self-example learning-guided single image super-resolution reconstruction method provided by the present invention in conjunction with the accompanying drawings, so that those skilled in the art can better understand the present invention and implement it.
[0093] The self-example is highly correlated with the input image, and the super-resolution image reconstruction method based on self-example learning has a better restoration effect on the internal texture features of the image. Compared with other learning-based methods, it is different for different super-resolution multiples and The image to be reconstructed has better adaptability. Since there are a large number of complex texture features in the original image, all the details of the image cannot be well reconstructed due to the limitation of the number of samples. Expanding the search space for similar image blocks in the image is a proble...
PUM
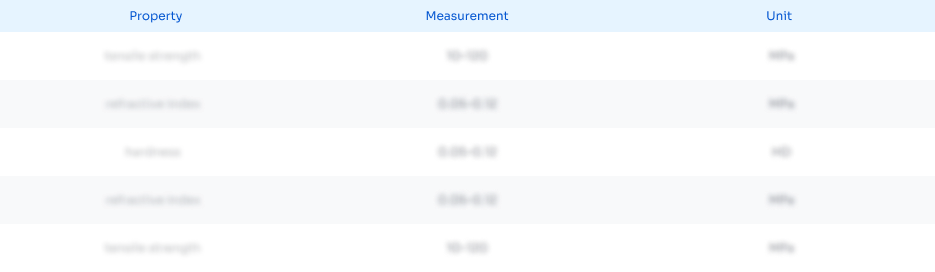
Abstract
Description
Claims
Application Information
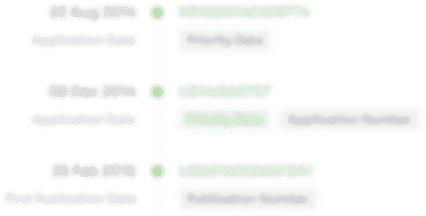
- Generate Ideas
- Intellectual Property
- Life Sciences
- Materials
- Tech Scout
- Unparalleled Data Quality
- Higher Quality Content
- 60% Fewer Hallucinations
Browse by: Latest US Patents, China's latest patents, Technical Efficacy Thesaurus, Application Domain, Technology Topic, Popular Technical Reports.
© 2025 PatSnap. All rights reserved.Legal|Privacy policy|Modern Slavery Act Transparency Statement|Sitemap|About US| Contact US: help@patsnap.com