Remote sensing scene classification method based on branch feature fusion convolutional network
A convolutional network and feature fusion technology, applied in scene recognition, biological neural network models, instruments, etc., can solve problems such as low complexity, high complexity, and complex spatial structure of shallow CNN models, and improve classification accuracy , the effect of great competitiveness
- Summary
- Abstract
- Description
- Claims
- Application Information
AI Technical Summary
Problems solved by technology
Method used
Image
Examples
specific Embodiment approach 1
[0016] Specific implementation mode 1: The specific process of the remote sensing scene classification method based on branch feature fusion convolutional network in this implementation mode is as follows:
[0017] In recent years, various researchers have made many effective attempts in the research work of remote sensing scene classification, and have proposed a large number of different classification methods. These works can be mainly divided into three categories, one is based on handcrafted feature (Handcrafted Feature-Based Methods), one is based on unsupervised feature learning (Unsupervised Feature-Learning-Based Methods), and finally as the current The mainstream CNN-based deep feature learning (Deep CNN Feature-Learning-Based Methods) method [22]. The relevant work of these three types of methods will be briefly introduced below, and then the main contributions of the work of the present invention will be given.
[0018] A. Handcrafted Feature-Based Approaches
[...
specific Embodiment approach 2
[0033] Specific embodiment two: the difference between this embodiment and specific embodiment one is: the LCNN-BFF network model is established in the step one; specifically:
[0034] LCNN-BFF model consists of input layer, batch normalization layer, ReLU activation layer, Group 1, Group 2, Group3, Group 4, Group 5, Group 6, Group 7, Group 8, Group9;
[0035] Group 1 includes the first regular convolution layer, the first depth separable convolution layer, batch normalization layer, ReLU activation layer and the first maximum pooling layer;
[0036] The size of the convolution kernel of the first conventional convolution layer and the first depth-separable convolution layer is 3×3, the number of convolution output channels is 32, and the convolution stride is 1; the pooling size of the first maximum pooling layer is 2×2, and the pooling stride is 2;
[0037] The output data of the input layer is input to the first regular convolutional layer, the output data of the first reg...
specific Embodiment approach 3
[0086] Embodiment 3: This embodiment differs from Embodiment 1 or Embodiment 2 in that: the input layer inputs remote sensing scene image data with a size of 256×256×3.
[0087] Other steps and parameters are the same as those in Embodiment 1 or Embodiment 2.
PUM
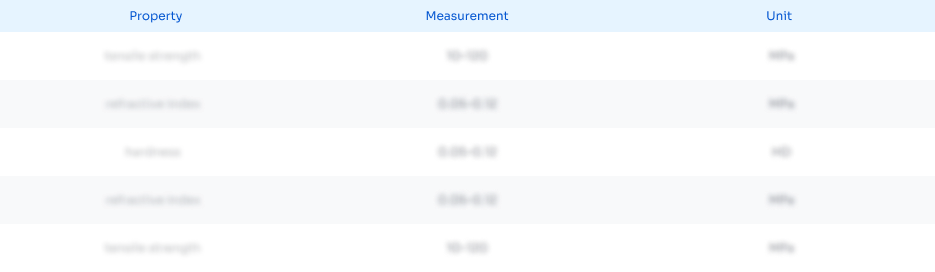
Abstract
Description
Claims
Application Information
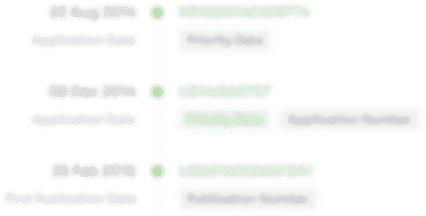
- R&D Engineer
- R&D Manager
- IP Professional
- Industry Leading Data Capabilities
- Powerful AI technology
- Patent DNA Extraction
Browse by: Latest US Patents, China's latest patents, Technical Efficacy Thesaurus, Application Domain, Technology Topic, Popular Technical Reports.
© 2024 PatSnap. All rights reserved.Legal|Privacy policy|Modern Slavery Act Transparency Statement|Sitemap|About US| Contact US: help@patsnap.com