Multi-sensor numerical control machine tool cutter wear monitoring method based on deep learning
A technology for CNC machine tools and tool wear, which is applied to manufacturing tools, measuring/indicating equipment, metal processing machinery parts, etc. It can solve the problems of inability to fuse sensor signals, low state recognition accuracy, and low efficiency, and achieve recognition accuracy. and the effect of recognition efficiency, reduced transition fitting, reduced dependence
- Summary
- Abstract
- Description
- Claims
- Application Information
AI Technical Summary
Problems solved by technology
Method used
Image
Examples
Embodiment Construction
[0033] The following will clearly and completely describe the technical solutions in the embodiments of the present invention with reference to the accompanying drawings in the embodiments of the present invention. Obviously, the described embodiments are only some, not all, embodiments of the present invention. Based on the embodiments of the present invention, all other embodiments obtained by persons of ordinary skill in the art without making creative efforts belong to the protection scope of the present invention.
[0034] In order to make the above objects, features and advantages of the present invention more comprehensible, the present invention will be further described in detail below in conjunction with the accompanying drawings and specific embodiments.
[0035] refer to figure 1 As shown, this embodiment provides a method for monitoring tool wear of a multi-sensor CNC machine tool based on deep learning, which specifically includes the following steps:
[0036] S...
PUM
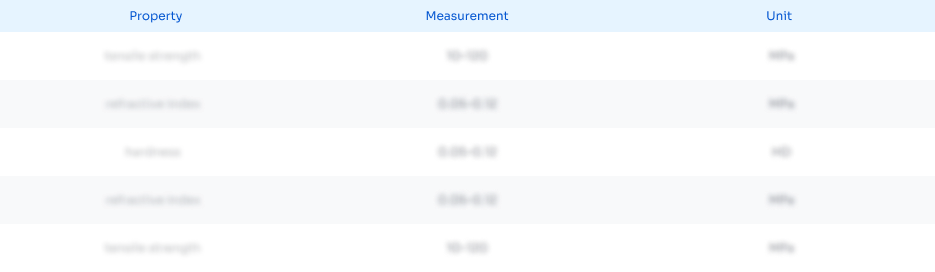
Abstract
Description
Claims
Application Information
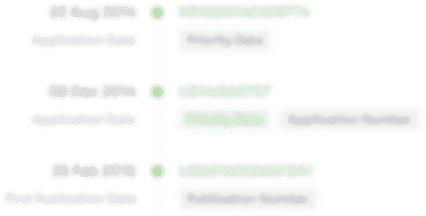
- R&D Engineer
- R&D Manager
- IP Professional
- Industry Leading Data Capabilities
- Powerful AI technology
- Patent DNA Extraction
Browse by: Latest US Patents, China's latest patents, Technical Efficacy Thesaurus, Application Domain, Technology Topic.
© 2024 PatSnap. All rights reserved.Legal|Privacy policy|Modern Slavery Act Transparency Statement|Sitemap