Video expression recognition method based on deep residual attention network
An expression recognition and attention technology, applied in neural learning methods, character and pattern recognition, biological neural network models, etc., can solve the problem of not taking into account the difference in the intensity of emotional representation of face images.
- Summary
- Abstract
- Description
- Claims
- Application Information
AI Technical Summary
Problems solved by technology
Method used
Image
Examples
Embodiment 1
[0048] Embodiment 1: A kind of video expression recognition method based on depth residual attention network of this embodiment, such as figure 1 shown, including the following steps:
[0049] S1. Perform video data preprocessing on video samples;
[0050] Step S1 comprises the following steps:
[0051] S1.1, first for each video sample, filter out the image frame of the peak intensity (apex) period;
[0052] S1.2, adopt haar-cascades detection model to carry out face detection; The face detection in the step S1.2 comprises the following steps:
[0053] Step 1. First convert the input image into a grayscale image to remove color interference;
[0054] Step 2. Set the size of the search face frame, search for faces in the input image in turn, find the faces and save them after intercepting;
[0055] Step 3. According to the standard distance between the two eyes, cut out images containing key expression parts such as mouth, nose, and forehead from the original facial expres...
PUM
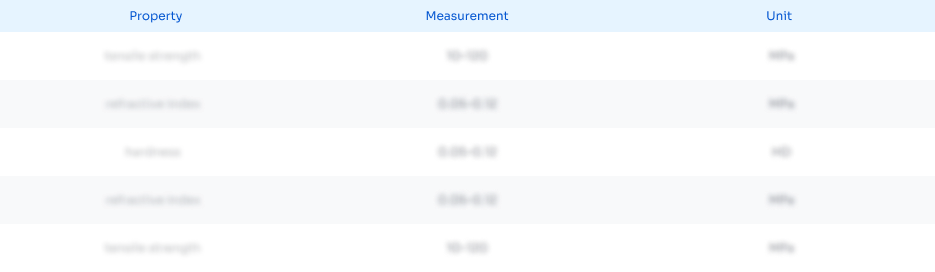
Abstract
Description
Claims
Application Information
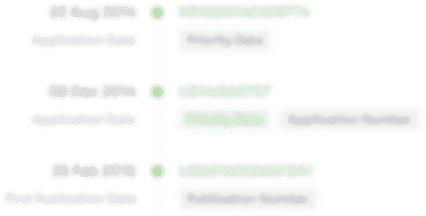
- R&D
- Intellectual Property
- Life Sciences
- Materials
- Tech Scout
- Unparalleled Data Quality
- Higher Quality Content
- 60% Fewer Hallucinations
Browse by: Latest US Patents, China's latest patents, Technical Efficacy Thesaurus, Application Domain, Technology Topic, Popular Technical Reports.
© 2025 PatSnap. All rights reserved.Legal|Privacy policy|Modern Slavery Act Transparency Statement|Sitemap|About US| Contact US: help@patsnap.com