Single-stage target detection method using anchor-frame-free module and enhanced classifier
A target detection and classifier technology, applied in the direction of instruments, character and pattern recognition, biological neural network models, etc., can solve problems that affect the accuracy of target positioning, missing objects, and detection algorithms that cannot be achieved
- Summary
- Abstract
- Description
- Claims
- Application Information
AI Technical Summary
Problems solved by technology
Method used
Image
Examples
Embodiment Construction
[0025] The technical solutions in the embodiments of the present invention are clearly and completely described. Obviously, the described embodiments are only some embodiments of the present invention, not all embodiments. Based on the embodiments of the present invention, all other embodiments obtained by persons of ordinary skill in the art without making creative efforts belong to the protection scope of the present invention.
[0026] Such as Figure 1-3 As shown, the present invention provides a technical solution: a single-stage target detection method using an anchor-free module and an enhanced classifier, including the following steps:
[0027] Embed the anchor-free frame module: the present invention uses the YOLOv3 model as the basic model, and selects CenterNet to realize the anchor-free frame module. The present invention directly uses DarkNet53 as the backbone network, and realizes three parallel sub-branches to generate prediction results. In order to save calcu...
PUM
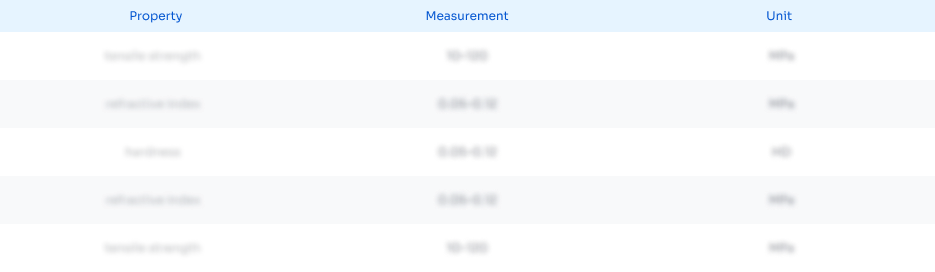
Abstract
Description
Claims
Application Information
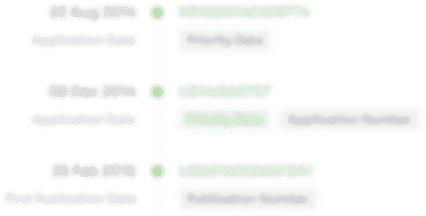
- R&D Engineer
- R&D Manager
- IP Professional
- Industry Leading Data Capabilities
- Powerful AI technology
- Patent DNA Extraction
Browse by: Latest US Patents, China's latest patents, Technical Efficacy Thesaurus, Application Domain, Technology Topic.
© 2024 PatSnap. All rights reserved.Legal|Privacy policy|Modern Slavery Act Transparency Statement|Sitemap