Deep transfer learning intelligent fault diagnosis method and device, storage medium and equipment
A technology of fault diagnosis and transfer learning, which is applied in the direction of neural learning methods, complex mathematical operations, character and pattern recognition, etc., can solve the problems of increased computing costs of MMD, and achieve the effect of improving the accuracy of transfer
- Summary
- Abstract
- Description
- Claims
- Application Information
AI Technical Summary
Problems solved by technology
Method used
Image
Examples
Embodiment 1
[0046] In this embodiment, a deep transfer learning intelligent fault diagnosis method includes:
[0047] Obtain the target domain data to be identified;
[0048] Input the target domain data into the fault diagnosis migration convolutional neural network model, and perform fault judgment on the target domain data through the fault diagnosis migration convolutional neural network model; wherein, the fault diagnosis migration convolutional neural network model is for The model obtained by the training process of the initial fault diagnosis migration convolutional neural network model.
[0049] Such as figure 1 As shown, the fault diagnosis migration convolutional neural network model includes a feature extractor and a health classifier; where the feature extractor includes a source domain feature extractor and a target domain feature extractor.
[0050] The source domain feature extractor includes sequentially connected convolutional layer 1S, pooling layer 1S, convolutional ...
Embodiment 2
[0094] In order to realize the deep transfer learning intelligent fault diagnosis method described in Embodiment 1, this embodiment provides a deep transfer learning intelligent fault diagnosis device, including:
[0095] A data input module, configured to obtain target domain data to be identified;
[0096] The data identification module is used to input the target domain data into the fault diagnosis migration convolutional neural network model, and perform fault judgment on the target domain data through the fault diagnosis migration convolutional neural network model; wherein, the fault diagnosis migration volume The product neural network model is a model obtained by training and processing the initial fault diagnosis migration convolutional neural network model;
[0097] The fault diagnosis migration convolutional neural network model is a model obtained by training and processing the initial fault diagnosis migration convolutional neural network model, which refers to: ...
Embodiment 3
[0099] A storage medium in this embodiment is characterized in that the storage medium stores a computer program, and when the computer program is executed by the processor, the processor executes the deep transfer learning intelligent fault diagnosis described in the first embodiment method.
PUM
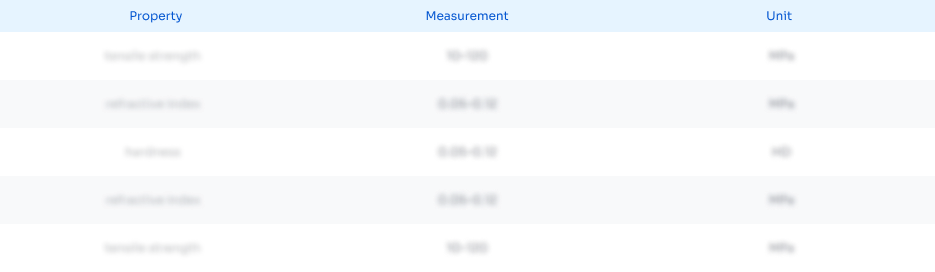
Abstract
Description
Claims
Application Information
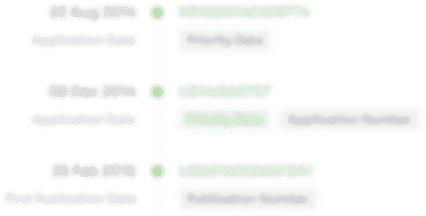
- R&D Engineer
- R&D Manager
- IP Professional
- Industry Leading Data Capabilities
- Powerful AI technology
- Patent DNA Extraction
Browse by: Latest US Patents, China's latest patents, Technical Efficacy Thesaurus, Application Domain, Technology Topic, Popular Technical Reports.
© 2024 PatSnap. All rights reserved.Legal|Privacy policy|Modern Slavery Act Transparency Statement|Sitemap|About US| Contact US: help@patsnap.com