Reinforcement learning method based on bidirectional model
A technology of reinforcement learning and forward model, applied in the field of reinforcement learning, to achieve the effect of small cumulative error of the model and excellent asymptotic performance
- Summary
- Abstract
- Description
- Claims
- Application Information
AI Technical Summary
Problems solved by technology
Method used
Image
Examples
Embodiment Construction
[0024] The following describes the preferred embodiments of the present application with reference to the accompanying drawings to make the technical content clearer and easier to understand. The present application can be embodied in many different forms of embodiments, and the protection scope of the present application is not limited to the embodiments mentioned herein.
[0025] The idea, specific structure and technical effects of the present invention will be further described below to fully understand the purpose, features and effects of the present invention, but the protection of the present invention is not limited thereto.
[0026] This embodiment is mainly used to solve the Mojoco robot control problem in the open source library Gym of OpenAI. Specifically, the definition state is the position and velocity of each part of the robot, and the action is the force applied to each part. The goal is to make the robot move as far as possible without falling down, and at th...
PUM
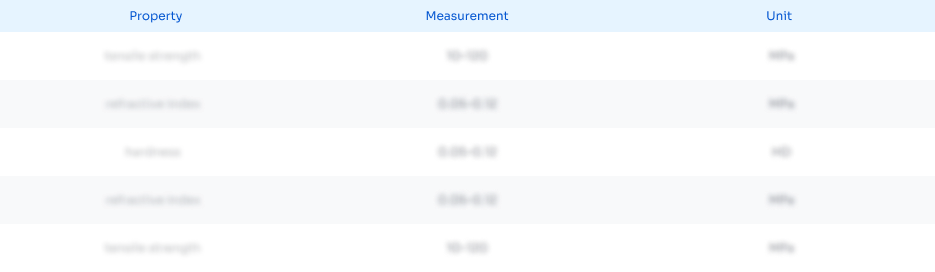
Abstract
Description
Claims
Application Information
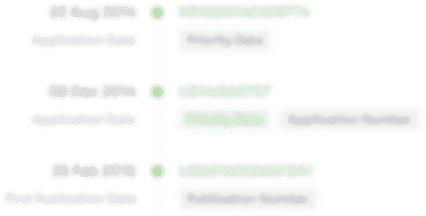
- R&D Engineer
- R&D Manager
- IP Professional
- Industry Leading Data Capabilities
- Powerful AI technology
- Patent DNA Extraction
Browse by: Latest US Patents, China's latest patents, Technical Efficacy Thesaurus, Application Domain, Technology Topic, Popular Technical Reports.
© 2024 PatSnap. All rights reserved.Legal|Privacy policy|Modern Slavery Act Transparency Statement|Sitemap|About US| Contact US: help@patsnap.com