Power load prediction method based on deep neural network
A deep neural network, power load technology, applied in the field of power load forecasting based on deep neural network, can solve the problems of incomplete collection of data, not considering holiday factors, etc.
- Summary
- Abstract
- Description
- Claims
- Application Information
AI Technical Summary
Problems solved by technology
Method used
Image
Examples
Embodiment
[0036] Embodiment: A kind of electric load forecasting method based on deep neural network of this embodiment comprises the following steps:
[0037] (1) Collect historical load data and use up to one week of historical load to predict the hourly load of a day in advance.
[0038] (2) Multiple parallel convolutional neural network (CNN) components are used to process historically loaded data, enabling the deep neural network model to automatically learn feature representations from raw data. Feature learning and feature extraction are performed in the first layer of the deep neural network model DNN, using a kernel with a locally connected receptive field, which acts as a filter for transforming the input signal, thus being able to learn various characteristics from the original input. At the same time, multiple parallel convolutional neural networks are used to transform the historical load sequence to obtain various features for subsequent load forecasting to obtain sequence d...
PUM
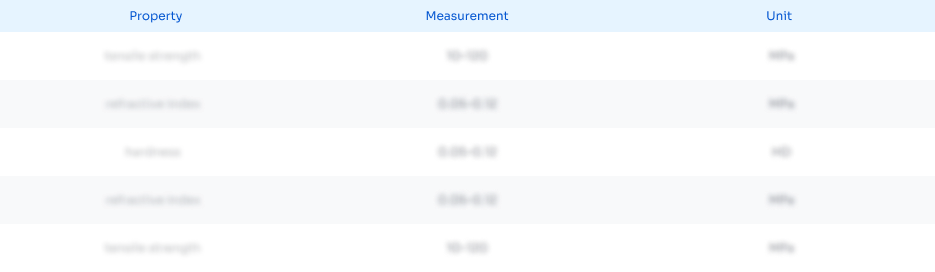
Abstract
Description
Claims
Application Information
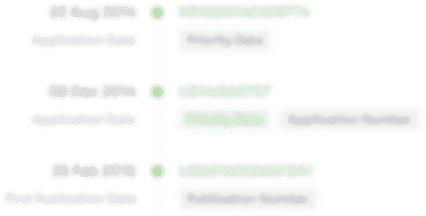
- R&D Engineer
- R&D Manager
- IP Professional
- Industry Leading Data Capabilities
- Powerful AI technology
- Patent DNA Extraction
Browse by: Latest US Patents, China's latest patents, Technical Efficacy Thesaurus, Application Domain, Technology Topic, Popular Technical Reports.
© 2024 PatSnap. All rights reserved.Legal|Privacy policy|Modern Slavery Act Transparency Statement|Sitemap|About US| Contact US: help@patsnap.com