Urban crowd flow prediction method based on long-term and short-term memory network model
A technology of long and short-term memory and network model, which is applied in the field of time series forecasting method - long and short-term memory network, which can solve the problems of poor forecasting effect and low forecasting accuracy on special holidays.
- Summary
- Abstract
- Description
- Claims
- Application Information
AI Technical Summary
Problems solved by technology
Method used
Image
Examples
Embodiment Construction
[0031] According to the principle of long short-term memory network, this design is further described in detail.
[0032] see figure 1 , figure 2 , the present invention is based on the long-short-term memory network model urban crowd flow prediction method is divided into the following five steps, each step is specifically as follows:
[0033] 1) Select input parameters according to the long short-term memory network model and output parameters
[0034] (1a) Input parameters: select the city's 3-year crowd flow as the observation sequence crowd flow data And the data values are normalized to [0,1] using min-max normalization, where k=i*365+j, Indicates the local population flow data of the city on the jth day of the i year, Indicates the city's out-of-town crowd flow data on the j-th day of the i-year, and k indicates the uniform sorting of the observation data by date. To group the data, the input of one input is:
[0035] (1b) Output parameter: Prediction ...
PUM
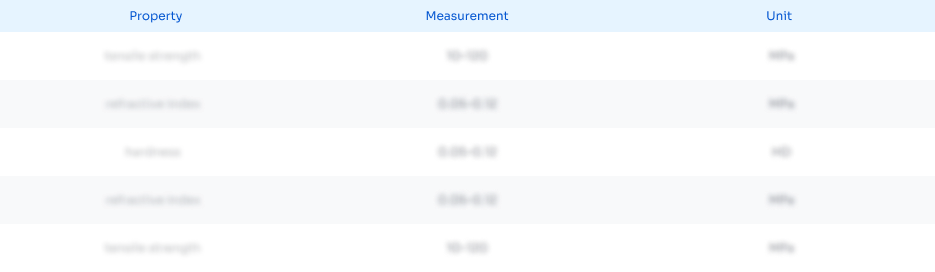
Abstract
Description
Claims
Application Information
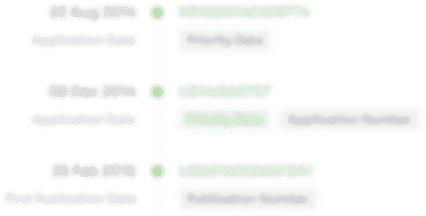
- R&D
- Intellectual Property
- Life Sciences
- Materials
- Tech Scout
- Unparalleled Data Quality
- Higher Quality Content
- 60% Fewer Hallucinations
Browse by: Latest US Patents, China's latest patents, Technical Efficacy Thesaurus, Application Domain, Technology Topic, Popular Technical Reports.
© 2025 PatSnap. All rights reserved.Legal|Privacy policy|Modern Slavery Act Transparency Statement|Sitemap|About US| Contact US: help@patsnap.com