Multi-task and cross-task supporting small sample classification training method and device
A small sample, multi-tasking technology, applied in character and pattern recognition, instruments, computer parts, etc., can solve problems such as lack of formal interpretation of perspective, inability to make full use of, and learning.
- Summary
- Abstract
- Description
- Claims
- Application Information
AI Technical Summary
Problems solved by technology
Method used
Image
Examples
Embodiment Construction
[0074] To address the shortcomings of applying deep learning techniques to small-shot classification, a common approach is to employ an auxiliary meta-learning or learned-to-learn model to train small-shot classification to learn a transferable good initial condition Or feature embedding, and then fine-tune the target small-sample classification problem through the learned optimization strategy or directly calculate and solve the target small-sample classification problem without updating the network weights. These meta-learning models have achieved important progress in few-shot classification. Among them, the most effective meta-learning model adopts an episode-based training framework. Each episode contains a small labeled support set and a corresponding query set to simulate the small sample setting in the test environment, thereby increasing the generalization of the model. ability. In this episode-based training framework, few-shot classification can be seen as the abil...
PUM
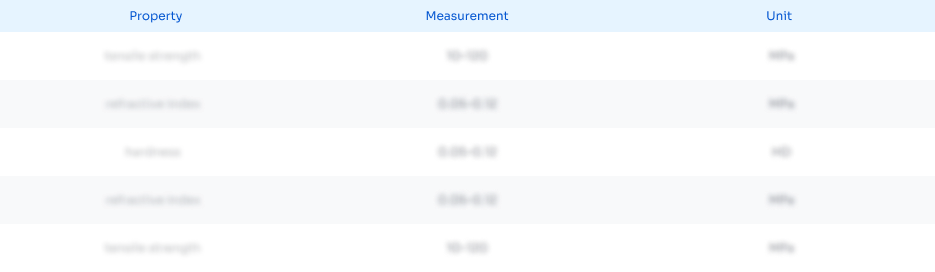
Abstract
Description
Claims
Application Information
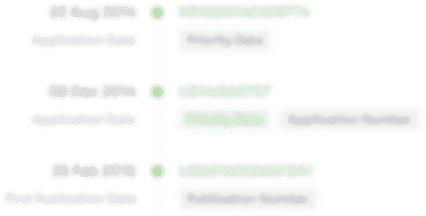
- R&D
- Intellectual Property
- Life Sciences
- Materials
- Tech Scout
- Unparalleled Data Quality
- Higher Quality Content
- 60% Fewer Hallucinations
Browse by: Latest US Patents, China's latest patents, Technical Efficacy Thesaurus, Application Domain, Technology Topic, Popular Technical Reports.
© 2025 PatSnap. All rights reserved.Legal|Privacy policy|Modern Slavery Act Transparency Statement|Sitemap|About US| Contact US: help@patsnap.com