Nonlinear 3DMM face reconstruction and posture normalization method and device, medium and equipment
A non-linear, normalized technology, applied in the field of computer vision, can solve problems such as faces that cannot handle large pose changes well
- Summary
- Abstract
- Description
- Claims
- Application Information
AI Technical Summary
Problems solved by technology
Method used
Image
Examples
Embodiment 1
[0096] Implementation of the present invention provides a nonlinear 3DMM face reconstruction method, such as figure 1 As shown, the method includes:
[0097] Step S100: use the training set to train the nonlinear 3DMM model.
[0098] Among them, the training set includes multiple 2D face image samples, and the nonlinear 3DMM model includes a CNN encoder, a multi-layer perception shape decoder, a CNN texture decoder, and a rendering layer.
[0099] The nonlinear 3DMM model of the present invention includes an encoder and two decoders, the encoder is a convolutional neural network, and the convolutional neural network (convolution neural network, CNN) is a deep learning method through which data can be extracted The deep-level feature descriptor, and the descriptor can be applied to tasks such as vision, speech and text. One of the two decoders is a shape decoder, the other is a texture decoder, the shape decoder is a deep convolutional neural network (CNN), the texture decode...
Embodiment 2
[0139] Embodiments of the present invention provide a nonlinear 3DMM face reconstruction device corresponding to the nonlinear 3DMM face reconstruction method described in Embodiment 1, such as Figure 5 As shown, the device includes:
[0140] The training module 10 is used to use the training set to train the nonlinear 3DMM model.
[0141] Among them, the training set includes multiple 2D face image samples, and the nonlinear 3DMM model includes a CNN encoder, a multi-layer perception shape decoder, a CNN texture decoder, and a rendering layer.
[0142] During training, the 2D face image sample input into the nonlinear 3DMM model is estimated by the CNN encoder to obtain the camera projection parameters, shape parameters and texture parameters. The multi-layer perception shape decoder decodes the shape parameters into 3D shapes, and the CNN texture decoder will The texture parameter is decoded into a 3D texture, and the rendering layer obtains the rendered image according to...
Embodiment 3
[0166] The methods described in the above embodiments provided in this specification can implement business logic through computer programs and record them on a storage medium, and the storage medium can be read and executed by a computer to achieve the effect of the solution described in Embodiment 1 of this specification. Therefore, the present invention also provides a computer-readable storage medium for nonlinear 3DMM face reconstruction corresponding to the nonlinear 3DMM face reconstruction method in Embodiment 1, including a memory for storing processor-executable instructions, the instruction When executed by the processor, the steps of the nonlinear 3DMM face reconstruction method in Embodiment 1 are realized.
[0167]In view of the obstacles of the existing linear 3DMM in its data, supervision and linear basis, the present invention learns a nonlinear 3DMM model of facial shape and texture from a set of unconstrained 2D face images by innovating the learning paradigm...
PUM
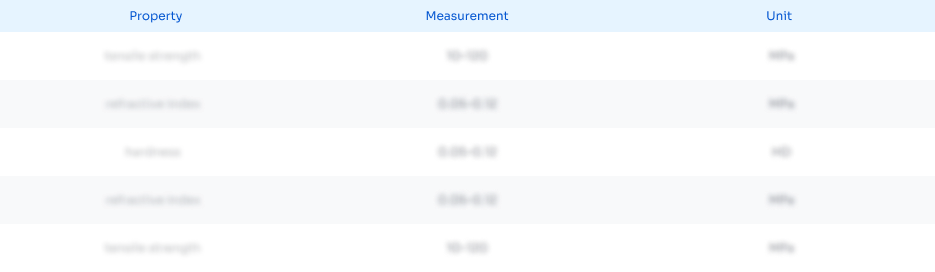
Abstract
Description
Claims
Application Information
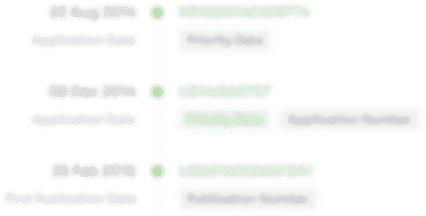
- R&D Engineer
- R&D Manager
- IP Professional
- Industry Leading Data Capabilities
- Powerful AI technology
- Patent DNA Extraction
Browse by: Latest US Patents, China's latest patents, Technical Efficacy Thesaurus, Application Domain, Technology Topic, Popular Technical Reports.
© 2024 PatSnap. All rights reserved.Legal|Privacy policy|Modern Slavery Act Transparency Statement|Sitemap|About US| Contact US: help@patsnap.com