Naive Bayes lithofacies classification ensemble learning method and device based on characteristic randomness
A classification integration and learning method technology, applied in the direction of integrated learning, computer components, special data processing applications, etc., can solve the problem of poor fitting effect of Gaussian distribution, complex and diverse real distribution of logging data, and accurate classification of lithofacies classifiers problems such as low accuracy, achieve good generalization performance, improve classification accuracy, and avoid time-consuming and labor-intensive effects
- Summary
- Abstract
- Description
- Claims
- Application Information
AI Technical Summary
Problems solved by technology
Method used
Image
Examples
Embodiment Construction
[0048] In order to enable those skilled in the art to better understand the technical solutions in this specification, the technical solutions in the embodiments of this specification will be clearly and completely described below in conjunction with the drawings in the embodiments of this specification. Obviously, the described The embodiments are only some of the embodiments in this specification, not all of the embodiments. Based on the implementations in this specification, all other implementations obtained by persons of ordinary skill in the art without creative efforts shall fall within the protection scope of this specification.
[0049] refer to figure 1 As shown, in some embodiments of this specification, the feature random-based naive Bayesian lithofacies classification ensemble learning method may include the following steps:
[0050] S101. Obtain various logging data of a target work area and perform preprocessing on them.
[0051] In the embodiment of this spec...
PUM
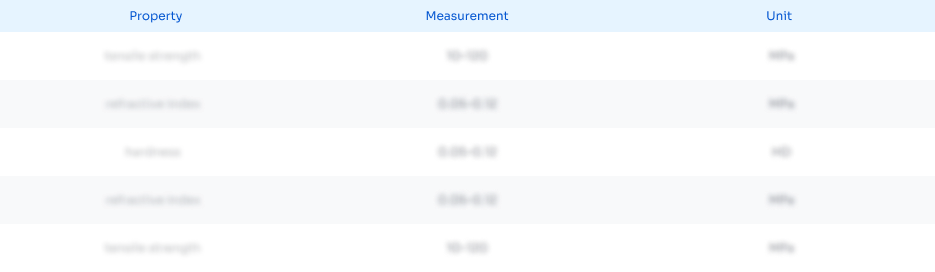
Abstract
Description
Claims
Application Information
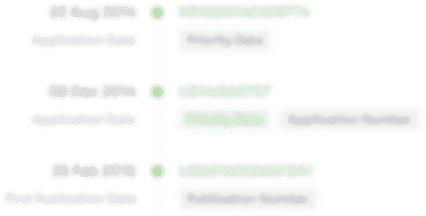
- R&D
- Intellectual Property
- Life Sciences
- Materials
- Tech Scout
- Unparalleled Data Quality
- Higher Quality Content
- 60% Fewer Hallucinations
Browse by: Latest US Patents, China's latest patents, Technical Efficacy Thesaurus, Application Domain, Technology Topic, Popular Technical Reports.
© 2025 PatSnap. All rights reserved.Legal|Privacy policy|Modern Slavery Act Transparency Statement|Sitemap|About US| Contact US: help@patsnap.com