Semantic segmentation method based on improved PSPNet
A semantic segmentation and model technology, applied in the field of computer vision, can solve problems such as inaccurate segmentation edges, affecting experimental results, and excessive parameters, and achieve the effects of reducing segmentation errors, improving segmentation accuracy, and reducing network parameters
- Summary
- Abstract
- Description
- Claims
- Application Information
AI Technical Summary
Problems solved by technology
Method used
Image
Examples
Embodiment Construction
[0031] The specific implementation manner of the present invention will be further described below in conjunction with the accompanying drawings.
[0032] figure 1 It is the overall network structure diagram of the present invention, including the feature extraction part; the context semantic feature supplement part; the pyramid pooling part; and predicting the category of each pixel. figure 2 is the process diagram of the level set method of the present invention, including the evolution result from the input image to the final one.
[0033] a) The present invention proposes: use a lightweight MobileNetV2 network to learn feature information. Introduce the contextual semantic feature supplementary module to retain more features, and introduce the level set method as the post-processing of the network after the network classifies and predicts each pixel in the image, so that the segmentation result is closer to the real contour of the target, and finally realizes the purpose...
PUM
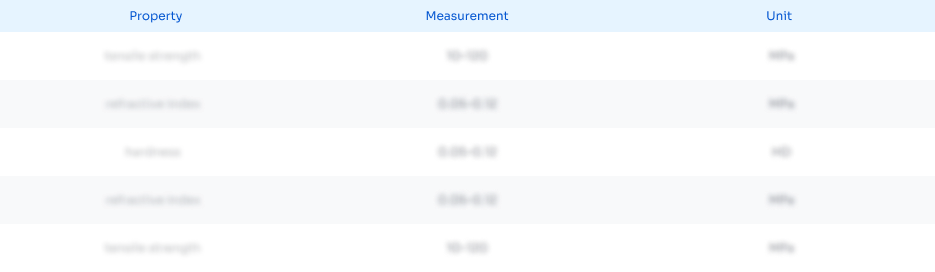
Abstract
Description
Claims
Application Information
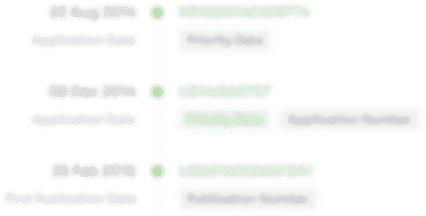
- R&D Engineer
- R&D Manager
- IP Professional
- Industry Leading Data Capabilities
- Powerful AI technology
- Patent DNA Extraction
Browse by: Latest US Patents, China's latest patents, Technical Efficacy Thesaurus, Application Domain, Technology Topic, Popular Technical Reports.
© 2024 PatSnap. All rights reserved.Legal|Privacy policy|Modern Slavery Act Transparency Statement|Sitemap|About US| Contact US: help@patsnap.com