Novel congestion control method combining deep reinforcement learning and traditional congestion control
A technology of congestion control and reinforcement learning, which is applied in network traffic/resource management, electrical components, wireless communication, etc., and can solve the problem that the communication network transmission layer congestion control strategy cannot take into account high performance, adaptability, fairness, and convergence. , to achieve the effect of flexibly adapting to application requirements, high practical value, and improving fairness/convergence
- Summary
- Abstract
- Description
- Claims
- Application Information
AI Technical Summary
Problems solved by technology
Method used
Image
Examples
Embodiment Construction
[0026] The present invention is described in further detail now in conjunction with accompanying drawing.
[0027] It should be noted that terms such as "upper", "lower", "left", "right", "front", and "rear" quoted in the invention are only for clarity of description, not for Limiting the practicable scope of the present invention, and the change or adjustment of the relative relationship shall also be regarded as the practicable scope of the present invention without substantive changes in the technical content.
[0028] The present invention is a novel congestion control method combining deep reinforcement learning and traditional congestion control (CUBIC), which mainly includes the following steps:
[0029] Step 1: The transport layer protocol obtains the performance preference defined by the actual application according to the requirements, and delivers it to the deep reinforcement learning module of the new congestion control algorithm; the new congestion control algorit...
PUM
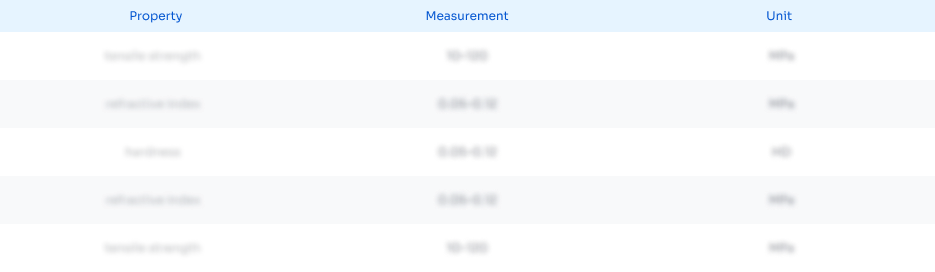
Abstract
Description
Claims
Application Information
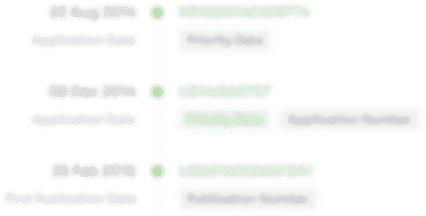
- R&D
- Intellectual Property
- Life Sciences
- Materials
- Tech Scout
- Unparalleled Data Quality
- Higher Quality Content
- 60% Fewer Hallucinations
Browse by: Latest US Patents, China's latest patents, Technical Efficacy Thesaurus, Application Domain, Technology Topic, Popular Technical Reports.
© 2025 PatSnap. All rights reserved.Legal|Privacy policy|Modern Slavery Act Transparency Statement|Sitemap|About US| Contact US: help@patsnap.com