Hyperspectral space-spectrum joint feature extraction method based on transfer learning
A technology of combined feature and transfer learning, applied in neural learning methods, instruments, biological neural network models, etc., can solve the problems of few data sets, high labeling costs, long training time, etc., to achieve good classification performance and improve classification accuracy. , the expression of accurate effect
- Summary
- Abstract
- Description
- Claims
- Application Information
AI Technical Summary
Problems solved by technology
Method used
Image
Examples
Embodiment Construction
[0034] The present invention will be further described in detail below in conjunction with the accompanying drawings and specific implementation examples.
[0035] A hyperspectral space-spectrum joint feature extraction method based on transfer learning, the steps are:
[0036] S1: Represent raw hyperspectral data as I ∈ R M×N×L , where I represents each sample in the data cube captured by the hyperspectral image sensor, M is the height, N is the width, and L represents the spectrum; before neural network model training, the original hyperspectral image is preprocessed first, using The spectral channel performs principal component analysis (PCA) operation to reduce the dimension of hyperspectral data to reduce the calculation cost; through PCA, the number of spectral channels is reduced from L to k, and the spatial size is kept unchanged; after PCA preprocessing, the The data is represented as I∈R M×N×k , where k is the number of principal components, where the value of k is...
PUM
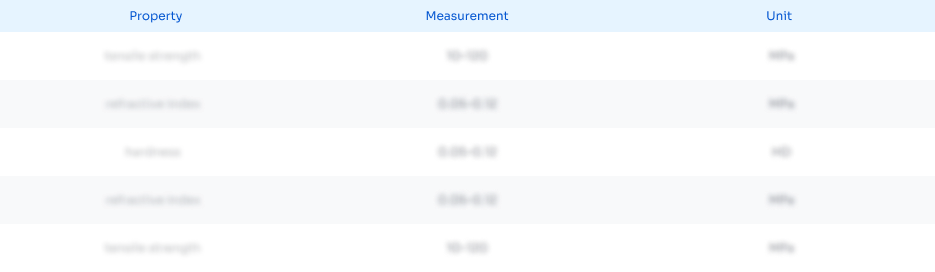
Abstract
Description
Claims
Application Information
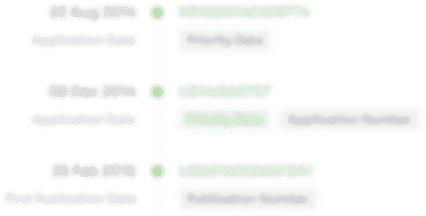
- R&D Engineer
- R&D Manager
- IP Professional
- Industry Leading Data Capabilities
- Powerful AI technology
- Patent DNA Extraction
Browse by: Latest US Patents, China's latest patents, Technical Efficacy Thesaurus, Application Domain, Technology Topic, Popular Technical Reports.
© 2024 PatSnap. All rights reserved.Legal|Privacy policy|Modern Slavery Act Transparency Statement|Sitemap|About US| Contact US: help@patsnap.com