Self-optimization decision control system of electric submersible pump
A technology of control system and electric submersible pump, applied in the direction of general control system, control/regulation system, adaptive control, etc., can solve the changeable and complex submersible electric pump that does not consider the environmental problems of injection and production The basic needs of intelligent control and other issues
- Summary
- Abstract
- Description
- Claims
- Application Information
AI Technical Summary
Problems solved by technology
Method used
Image
Examples
Embodiment 1
[0038] This embodiment provides a self-optimizing decision-making control system for electric submersible pumps, including an acquisition module 100 , a control module 200 , and an execution module 300 . Preferably, a collection module 100 for interacting with the environment is arranged in the injection-production wellbore. The acquisition module 100 is arranged downhole. The collection module 100 is capable of collecting environmental states. The environment is the entire drainage system including the reservoir. Environmental status includes reservoir information and drainage system information. For example, downhole flow rate, temperature, pressure, water content, liquid level height, liquid volume, electrical parameters and the diameter of the wellhead nozzle 402 for drainage by the electric submersible pump 400 . The electrical parameters include the parameters of the submersible motor 401, such as the frequency, voltage, and power consumption of the motor. Preferably...
Embodiment 2
[0091] This embodiment is a further improvement / supplement to Embodiment 1, and repeated content will not be repeated.
[0092] In Example 1, reinforcement learning is used for learning training and optimization decision-making, but the optimization decision in Example 1 is based on the fact that the state space S and action space A are discrete and the data dimension is small, which can achieve better convergence. However, the decision variable of the present invention, that is, the action space A is not a column vector, and not only the frequency of the submersible motor 401 but also the valve opening of the wellhead nozzle 402 are considered. Moreover, in actual situations, the number n of single wells may be large. In this case, it is unrealistic to store a large number of states and values corresponding to actions in the value table in reinforcement learning. Therefore, this embodiment combines deep learning on the basis of Embodiment 1 to solve the problem that the sta...
Embodiment 3
[0100] This embodiment is a further improvement / supplement to Embodiments 1 and 2 and their combination, and the repeated content will not be repeated.
[0101] The start-stop times, the first time and the second time in the thinning system in Embodiments 1 and 2 are fixed. Although production with this constant interval pumping system facilitates field management in the oilfield, the natural energy of the downhole formation of each single well is different, and the rate of rise and fall of the dynamic fluid level in the annulus is also different. Therefore, fixed first and second times do not apply to all single wells. Moreover, in the actual production process, the natural energy of the reservoir decreases continuously with the increase of the production time, and the corresponding thinning system should change dynamically.
[0102] On the other hand, if the number of starts and stops, the first time and the second time change dynamically, this makes the optimization decisi...
PUM
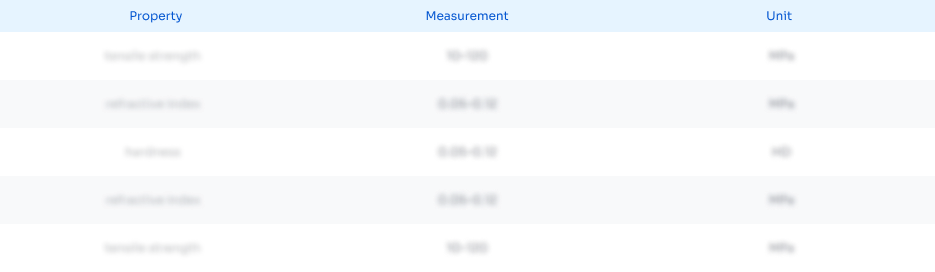
Abstract
Description
Claims
Application Information
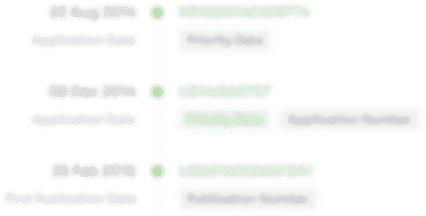
- R&D Engineer
- R&D Manager
- IP Professional
- Industry Leading Data Capabilities
- Powerful AI technology
- Patent DNA Extraction
Browse by: Latest US Patents, China's latest patents, Technical Efficacy Thesaurus, Application Domain, Technology Topic, Popular Technical Reports.
© 2024 PatSnap. All rights reserved.Legal|Privacy policy|Modern Slavery Act Transparency Statement|Sitemap|About US| Contact US: help@patsnap.com