Traffic prediction method based on multi-scale graph convolutional network model
A convolutional network and traffic forecasting technology, applied in biological neural network models, traffic flow detection, traffic control systems of road vehicles, etc., can solve poor forecasting results, ignore spatial dependence, and forecast results cannot reflect changes in traffic data Affected by the actual constraints of the urban road network and other issues, the prediction accuracy is high and the prediction effect is improved
- Summary
- Abstract
- Description
- Claims
- Application Information
AI Technical Summary
Problems solved by technology
Method used
Image
Examples
Embodiment Construction
[0025] Below in conjunction with accompanying drawing, further describe the present invention through embodiment, but do not limit the scope of the present invention in any way.
[0026] as attached figure 1 As shown, the flow of the traffic prediction method based on the multi-scale graph convolutional network model provided by the present invention includes:
[0027] 1) Preprocessing the collected raw traffic data;
[0028] 2) Construct a traffic network topology diagram according to the connection attributes of the urban traffic network;
[0029] 3) Standardize the adjacency matrix;
[0030] 4) Obtain the spatial dependence of the road network through a multi-scale graph convolutional network;
[0031] 5) Obtain the time dependence of the road network through the gated recurrent unit;
[0032] 6) Generate traffic flow forecasts through a linear transformation layer;
[0033] 7) Calculate the loss of actual traffic flow and predicted value;
[0034] 8) Carry out model ...
PUM
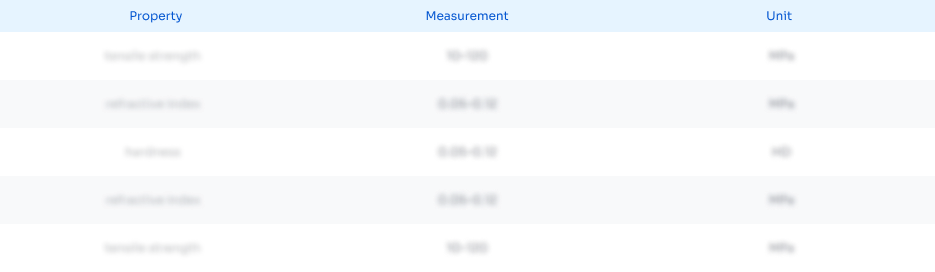
Abstract
Description
Claims
Application Information
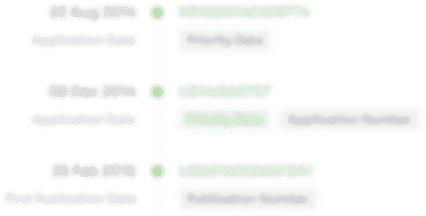
- R&D
- Intellectual Property
- Life Sciences
- Materials
- Tech Scout
- Unparalleled Data Quality
- Higher Quality Content
- 60% Fewer Hallucinations
Browse by: Latest US Patents, China's latest patents, Technical Efficacy Thesaurus, Application Domain, Technology Topic, Popular Technical Reports.
© 2025 PatSnap. All rights reserved.Legal|Privacy policy|Modern Slavery Act Transparency Statement|Sitemap|About US| Contact US: help@patsnap.com