Commodity recommendation model for relieving data sparsity and commodity cold start
A product recommendation, data sparse technology, applied in the field of interest mining, can solve the problems of product cold start, data sparsity, etc., to achieve the effect of enhancing expression ability, improving accuracy, and improving model performance
- Summary
- Abstract
- Description
- Claims
- Application Information
AI Technical Summary
Problems solved by technology
Method used
Image
Examples
Embodiment 1
[0067] In the product recommendation system, there are often two situations, the user has only interacted with a few products and the newly launched product has no user behavior, which are called sparsity and cold start problems respectively. For these two types of problems, the prediction performance of conventional recommendation models is not very good. Therefore, in the process of modeling product similarity through user behavior sequences in this application, EGES is used to embed and fuse various auxiliary information of products. , which alleviates the sparsity and cold start problems to a large extent.
[0068] Since the user's interest will change over a long period of time, while the interest in a short period of time is usually consistent, the sequence of product interaction time intervals of more than one hour is cut. Extract the user's historical behavior sequence, and construct a directed weighted graph G={V,E} through the obtained sequence, where the vertex V is...
Embodiment 2
[0078] In the existing technology, Google's Transformer model first proposed a multi-head attention mechanism (Multi-head Attention) and successfully applied it to machine translation, achieving remarkable results. The essence of the multi-head attention mechanism is to perform the Scaled Dot-Product Attention operation in the self-attention mechanism H times, and then obtain the output result through linear transformation. The benefit of the self-attention mechanism is that it can more effectively capture the long-distance interdependent features in the sequence, and the multi-head mechanism helps the network to capture richer feature information. Therefore, this application introduces this mechanism into the product recommendation scenario, and can better extract the dependencies between products for longer user behavior sequences.
[0079] First of all, since the self-attention module does not contain any loop or convolution structure, it is impossible to capture the order ...
Embodiment 3
[0096] In the actual e-commerce shopping scene, users’ interests usually migrate very quickly. We can effectively extract the dependencies between products through the multi-head self-attention network. The overall recommendation is based on all the user’s purchase history, not the next time. Buy recommended. Therefore, we also need to screen more influential user history behavior sequences for different candidate products.
[0097] The user behavior sequence is a time-related sequence, in which there are shallow or deep dependencies, and the recurrent neural network RNN and its variants have excellent performance for time series modeling, so the present invention proposes a A GRU (GRU with Attentional Update gate, AUGRU) structure based on the attention update gate, which more specifically simulates the interest evolution path related to candidate products, and also combines the attention mechanism to screen the interest evolution path .
[0098] The method of screening i...
PUM
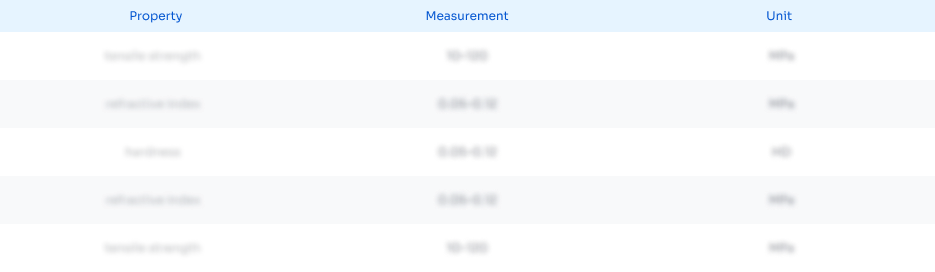
Abstract
Description
Claims
Application Information
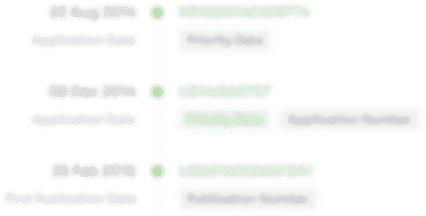
- R&D Engineer
- R&D Manager
- IP Professional
- Industry Leading Data Capabilities
- Powerful AI technology
- Patent DNA Extraction
Browse by: Latest US Patents, China's latest patents, Technical Efficacy Thesaurus, Application Domain, Technology Topic, Popular Technical Reports.
© 2024 PatSnap. All rights reserved.Legal|Privacy policy|Modern Slavery Act Transparency Statement|Sitemap|About US| Contact US: help@patsnap.com