Attribute graph literature clustering method based on graph convolutional neural network
A technology of convolutional neural network and document clustering, applied in neural learning methods, biological neural network models, still image data clustering/classification, etc. Effect
- Summary
- Abstract
- Description
- Claims
- Application Information
AI Technical Summary
Problems solved by technology
Method used
Image
Examples
Embodiment Construction
[0053] This paper takes Cora, Citeseer and Pubmed as examples to verify the effectiveness of the method. Firstly, the document attribute graph data is constructed with the above three databases. Document attribute graph can be expressed as G=(A,X), where A is an adjacency matrix, if document v i with v j There is a reference relationship between them, then A ij = 1, otherwise A ij =0. X is the document attribute matrix, the i-th row vector x in X i contains references to literature v i A description of the content. The construction method of X is as follows: (1) Eliminate function words in document documents, namely adverbs, prepositions, conjunctions, auxiliary words, etc. (2) Eliminate words whose frequency is less than 10. (3) Construct the word vector feature of each document with the remaining vocabulary, if the jth vocabulary is in the document v i appears in , then x ij = 1, otherwise x ij =0. The parameters of the constructed document attribute map are as fo...
PUM
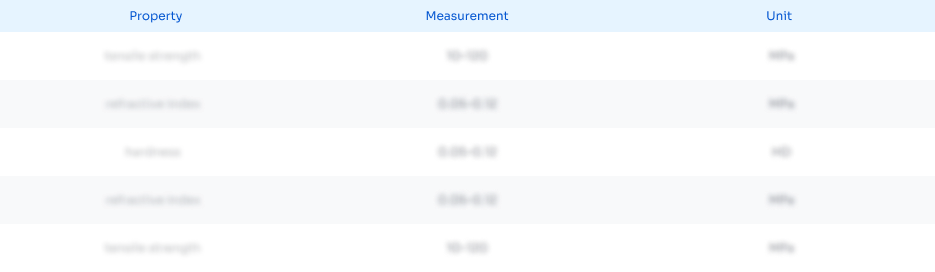
Abstract
Description
Claims
Application Information
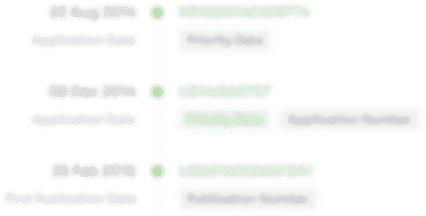
- R&D
- Intellectual Property
- Life Sciences
- Materials
- Tech Scout
- Unparalleled Data Quality
- Higher Quality Content
- 60% Fewer Hallucinations
Browse by: Latest US Patents, China's latest patents, Technical Efficacy Thesaurus, Application Domain, Technology Topic, Popular Technical Reports.
© 2025 PatSnap. All rights reserved.Legal|Privacy policy|Modern Slavery Act Transparency Statement|Sitemap|About US| Contact US: help@patsnap.com