Aircraft engine residual life prediction method based on deep learning coupling modeling
An aircraft engine, deep learning technology, applied in neural learning methods, stochastic CAD, biological neural network models, etc., can solve problems such as the inability to guarantee the fit of HI and failure process models, lack of internal connection, etc., to reduce economic and social losses. , the effect of accurate prediction
- Summary
- Abstract
- Description
- Claims
- Application Information
AI Technical Summary
Problems solved by technology
Method used
Image
Examples
Embodiment 1
[0060] This embodiment provides a method for predicting the remaining life of an aircraft engine based on deep learning coupling modeling, using the multiple sensor signal data collected during the operation of the aircraft engine to reflect its health status, to establish a deep learning coupling model, by combining DNN and LSTM Coupling, modeling the health state and failure process of the aircraft engine, and then realizing the prediction of the remaining life of the aircraft engine, such as figure 1 As shown, it specifically includes the following steps:
[0061] S1: Obtain the multi-element sensor failure signal of the aircraft engine;
[0062] S2: Load the pre-established and trained deep learning coupling model. The deep learning coupling model includes the interconnected failure process model LSTM and the fusion model DNN. The fusion model combines multi-sensor failure signals to construct the engine health index HI. The health index HI is defined as the potential fai...
PUM
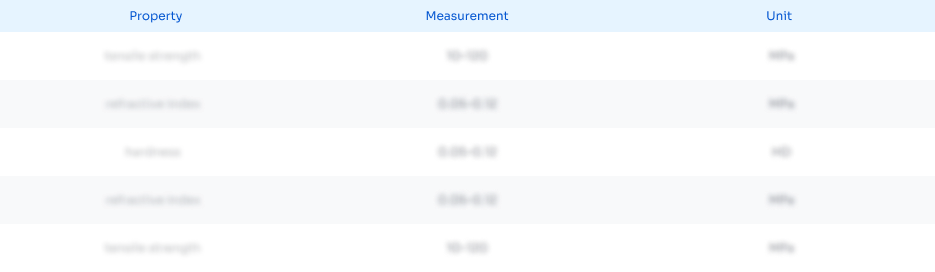
Abstract
Description
Claims
Application Information
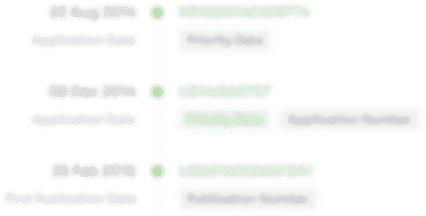
- R&D
- Intellectual Property
- Life Sciences
- Materials
- Tech Scout
- Unparalleled Data Quality
- Higher Quality Content
- 60% Fewer Hallucinations
Browse by: Latest US Patents, China's latest patents, Technical Efficacy Thesaurus, Application Domain, Technology Topic, Popular Technical Reports.
© 2025 PatSnap. All rights reserved.Legal|Privacy policy|Modern Slavery Act Transparency Statement|Sitemap|About US| Contact US: help@patsnap.com