Pedestrian re-identification method based on clustering guidance and paired measurement triple loss
A pedestrian re-identification and triplet technology, applied in the field of computer vision and pattern recognition, can solve the problems of increasing the computational complexity of the model, the difficulty of adjusting parameters in network training settings, the inability to solve the problems of too many outliers, and the incompatibility of neural networks, etc., to achieve Improve metric learning performance, improve recognition rate, improve convergence ability and performance effect
- Summary
- Abstract
- Description
- Claims
- Application Information
AI Technical Summary
Problems solved by technology
Method used
Image
Examples
Embodiment Construction
[0069] The present invention will be described in detail below in conjunction with the accompanying drawings and specific embodiments. The following description is only for demonstration and explanation, and does not limit the present invention in any form.
[0070] The general loss identification steps have been described in detail in the "Summary of the Invention", and the identification process of the present invention will be specifically described in conjunction with examples. figure 1 It is a schematic diagram of the overall processing flow.
[0071] Technical scheme of the present invention mainly comprises the steps:
[0072] Step 1. This step obtains a similarity label matrix based on the features output by the deep convolutional learning network and the labels corresponding to each feature sample in its training batch;
[0073] 1-1. The characteristics of the input deep learning network output Where b is the training batch, c is the tensor dimension, and the featu...
PUM
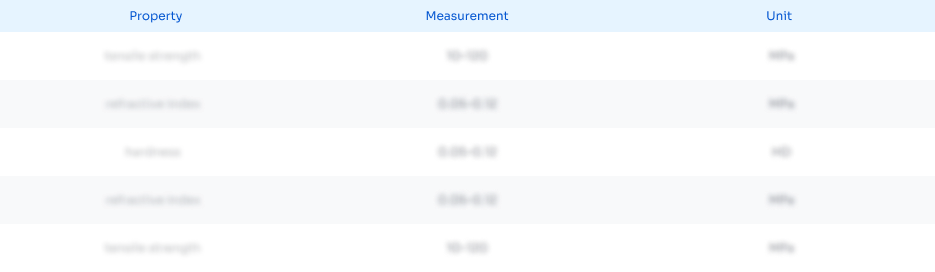
Abstract
Description
Claims
Application Information
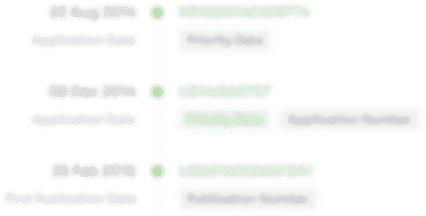
- Generate Ideas
- Intellectual Property
- Life Sciences
- Materials
- Tech Scout
- Unparalleled Data Quality
- Higher Quality Content
- 60% Fewer Hallucinations
Browse by: Latest US Patents, China's latest patents, Technical Efficacy Thesaurus, Application Domain, Technology Topic, Popular Technical Reports.
© 2025 PatSnap. All rights reserved.Legal|Privacy policy|Modern Slavery Act Transparency Statement|Sitemap|About US| Contact US: help@patsnap.com