Facial expression recognition method based on graph convolutional network
A convolutional network and facial expression technology, applied in the field of image processing and image recognition, can solve the problems of losing image information, selecting nodes with richer identification information, affecting the accuracy of face area image expression recognition, etc., to improve the accuracy , The effect of improving the accuracy of expression recognition
- Summary
- Abstract
- Description
- Claims
- Application Information
AI Technical Summary
Problems solved by technology
Method used
Image
Examples
Embodiment Construction
[0026] Combine below figure 1 , to further describe in detail the specific steps of the present invention.
[0027] Step 1, generate a training set.
[0028] The first step is to collect at least 5000 face pictures, each face picture contains 1 facial expression and the corresponding expression label, each person collects at least 7 kinds of expressions, and at least 2 pictures for each expression.
[0029] The second step is to use the 68 face key point detection algorithm to detect each face key point in each picture, and obtain the face key points containing the coordinate information of each face key point in each picture.
[0030] Described 68 people's face key points detection algorithm refers to, locates the people's face area in each picture of input; Utilizes the well-trained 68 people's faces key point feature detectors, extracts the transverse direction of 68 people's faces key points in the people's face area , the ordinate value.
[0031] In the third step, bas...
PUM
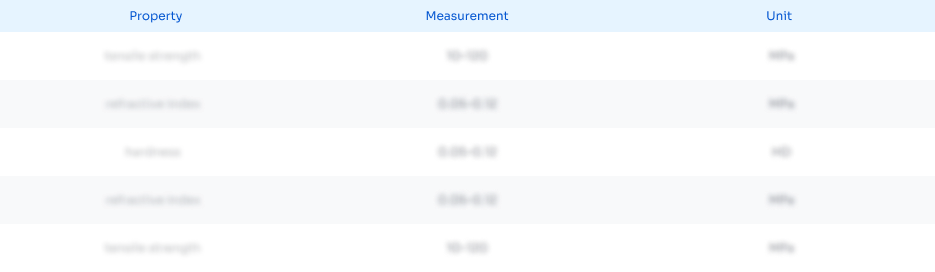
Abstract
Description
Claims
Application Information
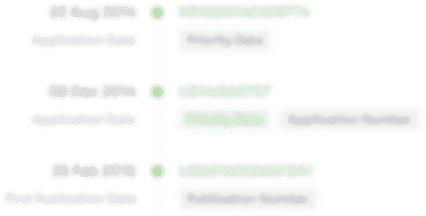
- R&D Engineer
- R&D Manager
- IP Professional
- Industry Leading Data Capabilities
- Powerful AI technology
- Patent DNA Extraction
Browse by: Latest US Patents, China's latest patents, Technical Efficacy Thesaurus, Application Domain, Technology Topic, Popular Technical Reports.
© 2024 PatSnap. All rights reserved.Legal|Privacy policy|Modern Slavery Act Transparency Statement|Sitemap|About US| Contact US: help@patsnap.com