Facial expression recognition method based on improved deep convolutional generative adversarial network
A technology of facial expression recognition and deep convolution, which is applied in the field of deep learning and image processing, can solve problems such as blurred images, uneven light in images, and reduced performance of the target database, so as to help identify and capture and overcome uneven light The effect of improving learning performance
- Summary
- Abstract
- Description
- Claims
- Application Information
AI Technical Summary
Problems solved by technology
Method used
Image
Examples
Embodiment Construction
[0051] In this embodiment, a facial expression recognition method based on an improved deep convolution generation confrontation network, such as figure 1 As shown, proceed as follows:
[0052] Step 1. Obtain the face image dataset and perform preprocessing:
[0053] Obtain the real face image dataset and crop them into face images with a size of M×M, and then randomly add mask to simulate the uneven light, image damage, face occlusion, etc. of the face image in reality, and the preprocessed face image data set is denoted as X={x 1 ,x 2 ,...,x i ,...,x N}, where x i Represent the i-th face image, i=1, 2,..., N, N is the total number of face images in the face image data set, in the present embodiment, select the face frontal picture in the VGGFace data set as the training data set, The collected images are uniformly cropped into 128×128 images.
[0054] Step 2. Build an improved deep convolutional generation confrontation network consisting of a generative network G an...
PUM
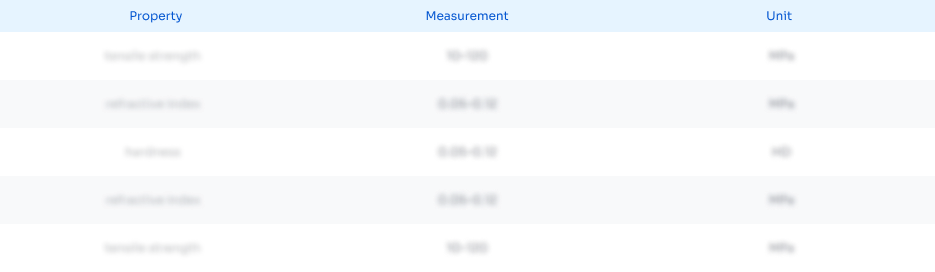
Abstract
Description
Claims
Application Information
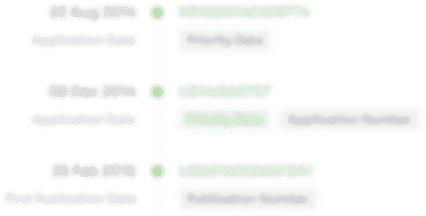
- R&D Engineer
- R&D Manager
- IP Professional
- Industry Leading Data Capabilities
- Powerful AI technology
- Patent DNA Extraction
Browse by: Latest US Patents, China's latest patents, Technical Efficacy Thesaurus, Application Domain, Technology Topic, Popular Technical Reports.
© 2024 PatSnap. All rights reserved.Legal|Privacy policy|Modern Slavery Act Transparency Statement|Sitemap|About US| Contact US: help@patsnap.com