Real world image super-resolution method and system based on degraded variational auto-encoder
An autoencoder and real-world technology, applied in the field of real-world image super-resolution method and system, can solve the problems of unstable training effect and unsatisfactory image super-resolution effect
- Summary
- Abstract
- Description
- Claims
- Application Information
AI Technical Summary
Problems solved by technology
Method used
Image
Examples
Embodiment 1
[0023] like figure 1 As shown, an embodiment of the present invention provides a real world image of a denominate variation from the encoder, including the following steps:
[0024] Step S1: Get the training data set and preprocess the data set to obtain a well-resolution image and high resolution image;
[0025] Step S2: Enter a low resolution image to obtain a corresponding high-definition image; enter the high-definition image input denometer from the encoding network to obtain a reconstructed low resolution image;
[0026] Step S3: Enter the high-resolution image input denometer from the encoding network to obtain a corresponding low-definition image; enter the low-definition image to a super-resolution network to obtain a high resolution image;
[0027] Step S4: Build a loop consistent loss function, according to the low-resolution image and the reconstructed low-resolution image, and the high-resolution image and the reconstructed high-resolution image calculate the loop con...
Embodiment 2
[0061] like Figure 5 Shown embodiment the present invention provides the following sub-module for a qualitative drop from the encoder real-world system is based on super-resolution image, comprising:
[0062] Training image acquiring module, for acquiring training data set, pre-processing and data sets, to obtain good low-resolution image processing and high resolution image;
[0063] Acquiring low resolution image reconstruction module for the super-resolution low-resolution image input network, to obtain high-definition images corresponding to; the HD image from the input coded sub-network qualitative drop, to obtain a low-resolution image reconstruction;
[0064] The reconstructed high resolution image acquisition module for the high resolution image from the input coded sub-network qualitative reduction to give clear images corresponding to low; low clearing the super-resolution image input network to obtain the reconstructed high-resolution image;
[0065] Training super-reso...
PUM
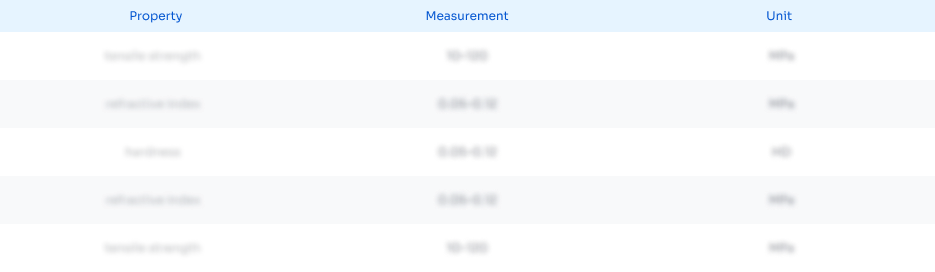
Abstract
Description
Claims
Application Information
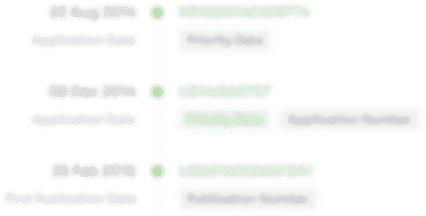
- R&D Engineer
- R&D Manager
- IP Professional
- Industry Leading Data Capabilities
- Powerful AI technology
- Patent DNA Extraction
Browse by: Latest US Patents, China's latest patents, Technical Efficacy Thesaurus, Application Domain, Technology Topic, Popular Technical Reports.
© 2024 PatSnap. All rights reserved.Legal|Privacy policy|Modern Slavery Act Transparency Statement|Sitemap|About US| Contact US: help@patsnap.com