Image super-resolution reconstruction method based on cascade residual convolutional neural network
A convolutional neural network and super-resolution reconstruction technology, which is used in video and image processing. In the field of high-resolution image reconstruction, residual convolutional neural network can solve the problem of PSNR value drop of reconstructed image, poor subjective perception effect of reconstructed image, etc. problem, to achieve the effect of accelerating the convergence speed and simplifying the training process
- Summary
- Abstract
- Description
- Claims
- Application Information
AI Technical Summary
Problems solved by technology
Method used
Image
Examples
Embodiment Construction
[0031] As a classic topology in artificial neural networks, convolutional neural networks have a wide range of applications in the fields of pattern recognition, image and speech information analysis and processing. In the field of image super-resolution reconstruction, after Dong Chao and others first proposed the SRCNN[4] network and successfully applied the convolutional neural network (CNN) to the restoration and reconstruction of high-resolution images, many improved CNNs have been successively adopted. proposed, and have achieved significant improvement in the key reconstruction effect evaluation indicators.
[0032] In order to obtain better reconstruction results, the existing super-resolution reconstruction methods of CNN-based images often have very deep network layers, resulting in too long network training time, slow convergence speed, and more prone to gradient disappearance or Gradient explosion and other problems increase the difficulty of network training. At ...
PUM
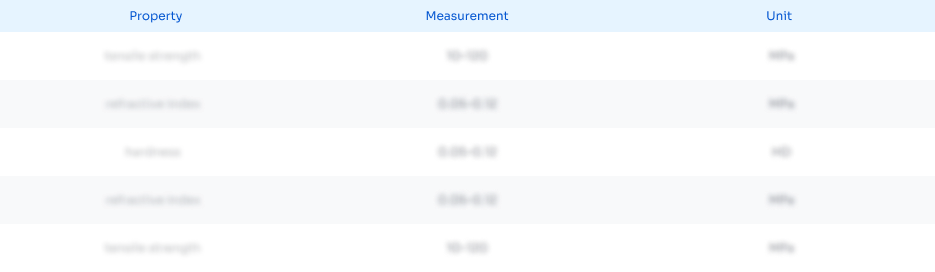
Abstract
Description
Claims
Application Information
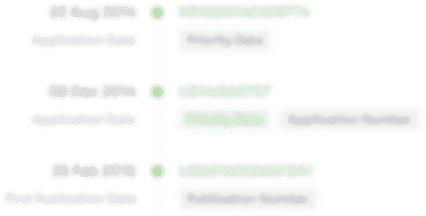
- R&D Engineer
- R&D Manager
- IP Professional
- Industry Leading Data Capabilities
- Powerful AI technology
- Patent DNA Extraction
Browse by: Latest US Patents, China's latest patents, Technical Efficacy Thesaurus, Application Domain, Technology Topic, Popular Technical Reports.
© 2024 PatSnap. All rights reserved.Legal|Privacy policy|Modern Slavery Act Transparency Statement|Sitemap|About US| Contact US: help@patsnap.com