Long-tail distribution image classification method with noise label
A classification method and labeling technology, applied in neural learning methods, instruments, biological neural network models, etc., can solve problems such as ignoring data distribution, and achieve the effect of increasing tolerance, improving performance, and reducing impact
- Summary
- Abstract
- Description
- Claims
- Application Information
AI Technical Summary
Problems solved by technology
Method used
Image
Examples
Embodiment Construction
[0042] Specific embodiments of the present invention will be described in detail below in conjunction with the accompanying drawings. It should be understood that the specific embodiments described here are only used to illustrate and explain the present invention, and are not intended to limit the present invention.
[0043] Noise label learning has received a lot of attention in recent years and has achieved amazing results. However, existing deep neural networks (DNNs) are still deficient in addressing noisy labels and long-tail learning. Such as Figure 1a shown, where the symmetric noise rate , when DNNs are used to fit noisy labels, fluctuations in validation accuracy explain the noise capacity of the model. Such as Figure 1b Shown, where the imbalance factor , the application of DNN in long-tail distribution learning also reflects similar characteristics, that is, DNN first fits the main category, and then gradually fits the tail category. From the above analysi...
PUM
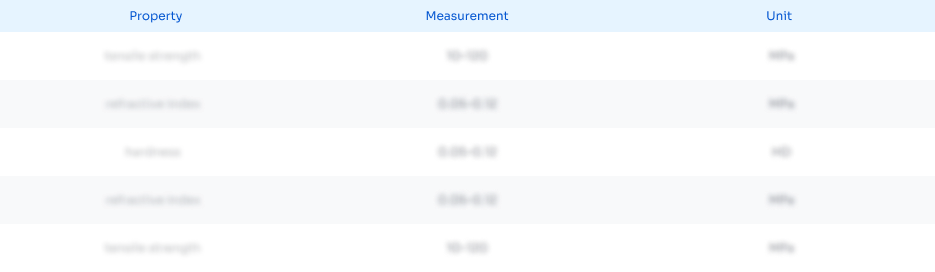
Abstract
Description
Claims
Application Information
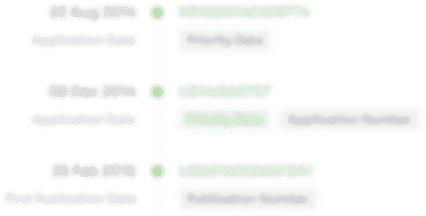
- R&D Engineer
- R&D Manager
- IP Professional
- Industry Leading Data Capabilities
- Powerful AI technology
- Patent DNA Extraction
Browse by: Latest US Patents, China's latest patents, Technical Efficacy Thesaurus, Application Domain, Technology Topic.
© 2024 PatSnap. All rights reserved.Legal|Privacy policy|Modern Slavery Act Transparency Statement|Sitemap