Prediction method for time sequence intervention effect
A prediction method and timing technology, applied in the field of data processing, can solve problems such as complex formation mechanism
- Summary
- Abstract
- Description
- Claims
- Application Information
AI Technical Summary
Problems solved by technology
Method used
Image
Examples
Embodiment Construction
[0044] The present invention will be specifically described below with reference to the accompanying drawings and specific examples.
[0045] The present invention discloses a prediction method of timing intervention effects, including the following steps:
[0046] S101: Get timing retrospective experimental data set, divide it into training set, verification set and test set.
[0047] Timing retrospective data set D is the number of samples, each sample in, Represents the characterization of the i-th sample in the tuals, interventions, and intervention effects, V (i) Represents static feature items with time change, L (i) Indicates the length of the sequence. In the subsequent statements, the number (I) will be omitted unless necessary. Remember the historical sequence of the sample in For the feature item sequence, For interventions sequence, T is the length of the historical sequence.
[0048] Further, feature item sequence Contains three partial hidden versions of each...
PUM
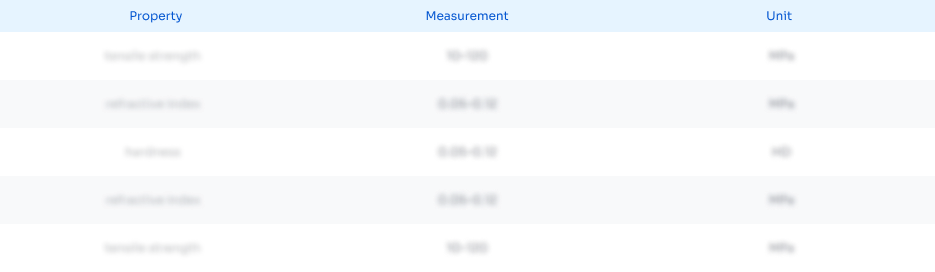
Abstract
Description
Claims
Application Information
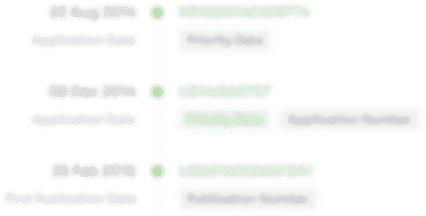
- R&D Engineer
- R&D Manager
- IP Professional
- Industry Leading Data Capabilities
- Powerful AI technology
- Patent DNA Extraction
Browse by: Latest US Patents, China's latest patents, Technical Efficacy Thesaurus, Application Domain, Technology Topic, Popular Technical Reports.
© 2024 PatSnap. All rights reserved.Legal|Privacy policy|Modern Slavery Act Transparency Statement|Sitemap|About US| Contact US: help@patsnap.com