Brain age assessment method for Rolandic epilepsy children based on machine learning
A machine learning, epilepsy technology, used in sensors, medical science, telemetry patient monitoring, etc.
- Summary
- Abstract
- Description
- Claims
- Application Information
AI Technical Summary
Problems solved by technology
Method used
Image
Examples
Embodiment 1
[0053] The basic method of the present invention to realize the brain age assessment of children with Rolandic epilepsy is:
[0054] S100. Collect the MRI data of the subjects in the control group, and perform effective model feature extraction through dimensionality reduction;
[0055] S200. Construct a prediction model based on the effective model features extracted in S100;
[0056] S300. Collect the MRI data of the epileptic child and extract the characteristics of the tested model, input the characteristics of the tested model into the prediction model and output the prediction result;
[0057] Wherein, the MRI data in S100 includes brain gray matter thickness, brain gray matter surface area, and brain gray matter volume, and the effective model features include at least one of the gray matter thickness, surface area of gray matter, and gray matter volume in each subdivided part index and mixed index.
[0058] see Figure 14 , the more specific implementation method i...
Embodiment 2
[0064] Referring to the more specific implementation method described in Example 1, this example will describe the implementation process in detail.
[0065] 1. Object selection
[0066] Fifty children with Rolandic epilepsy and 50 healthy control children matched in gender, age and years of education were selected.
[0067] Case group inclusion and exclusion criteria:
[0068] (1) Inclusion criteria:
[0069] 1) Meet the Rolandic epilepsy diagnosis;
[0070] 2) Age 6-16 years old;
[0071] (2) Exclusion criteria:
[0072] 1) Those with MRI contraindications and those with claustrophobia;
[0073] 2) Heart, lung and other vital organ failure;
[0074] 3) History of neuropsychiatric diseases other than Rolandic epilepsy, such as trauma, tumor, infection, etc.;
[0075] 4) Those with abnormal brain routine MRI examination;
[0076] 5) MRI image artifacts seriously affect image analysts.
[0077] Inclusion and exclusion criteria for the control group:
[0078] (1) Inclu...
PUM
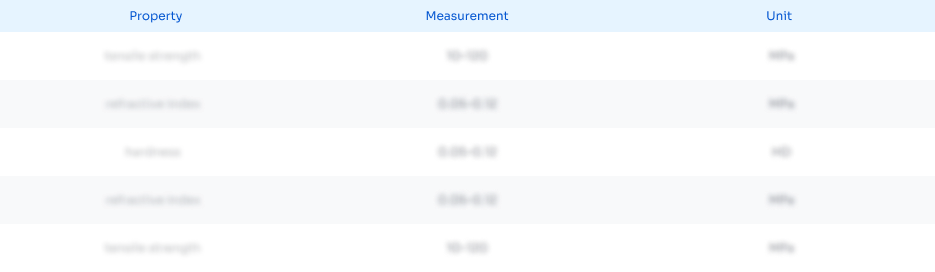
Abstract
Description
Claims
Application Information
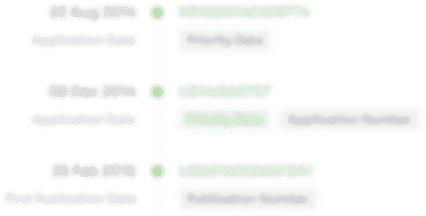
- Generate Ideas
- Intellectual Property
- Life Sciences
- Materials
- Tech Scout
- Unparalleled Data Quality
- Higher Quality Content
- 60% Fewer Hallucinations
Browse by: Latest US Patents, China's latest patents, Technical Efficacy Thesaurus, Application Domain, Technology Topic, Popular Technical Reports.
© 2025 PatSnap. All rights reserved.Legal|Privacy policy|Modern Slavery Act Transparency Statement|Sitemap|About US| Contact US: help@patsnap.com