Electronic fence risk early warning method based on deep learning
An electronic fence and deep learning technology, applied in the field of deep learning, can solve problems such as the difficulty in forming a unified integrated platform, the inability to provide open protocols and interfaces, and the control and management of the perimeter alarm system, so as to achieve the effect of avoiding impact
- Summary
- Abstract
- Description
- Claims
- Application Information
AI Technical Summary
Problems solved by technology
Method used
Image
Examples
Embodiment Construction
[0039] In order to make the object, technical solution and advantages of the present invention more clear, the present invention will be further described in detail below in conjunction with the accompanying drawings and embodiments. It should be understood that the specific embodiments described are only used to explain the present invention, but not to limit the present invention. Based on the embodiments of the present invention, all other embodiments obtained by ordinary persons in the art without creative efforts shall fall within the protection scope of the present invention.
[0040] Refer to attached figure 1 , the present invention provides a method for early warning of electronic fence risks based on deep learning, including:
[0041] Obtain an image containing a cone and mark its position, construct a cone detection model, and input the image of the marked cone position into the cone detection model for training;
[0042] Through the trained cone detection model, ...
PUM
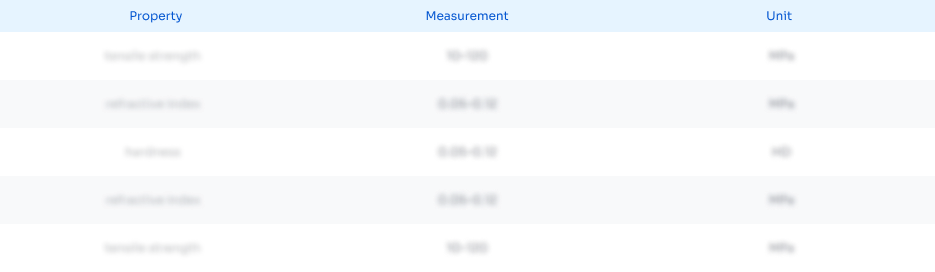
Abstract
Description
Claims
Application Information
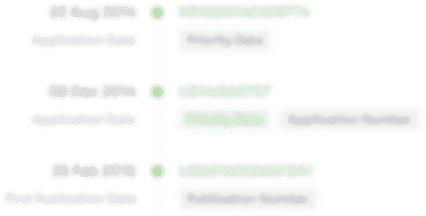
- R&D Engineer
- R&D Manager
- IP Professional
- Industry Leading Data Capabilities
- Powerful AI technology
- Patent DNA Extraction
Browse by: Latest US Patents, China's latest patents, Technical Efficacy Thesaurus, Application Domain, Technology Topic, Popular Technical Reports.
© 2024 PatSnap. All rights reserved.Legal|Privacy policy|Modern Slavery Act Transparency Statement|Sitemap|About US| Contact US: help@patsnap.com