Adversarial patch generation method
A technology of patches and calculation methods, applied in the field of machine learning, which can solve problems such as pixel value exceeding
- Summary
- Abstract
- Description
- Claims
- Application Information
AI Technical Summary
Problems solved by technology
Method used
Image
Examples
Embodiment
[0052] In the present invention, similar to Thys et al.'s method, they generate patches by optimizing the confidence of a specific category. However, the difference is that Thys et al.'s method selects all bounding The maximum confidence in the box (bounding box) is used as the objective function. Such an optimization strategy is difficult to guarantee that the maximum target confidence obtained is the confidence of the category to be targeted, so it is possible to optimize other The confidence of the bounding box of the category target, thereby affecting the detection results of other categories, and the present invention optimizes the confidence of all bounding boxes of the target of the category to be targeted, and optimizes it by using cross entropy as the objective function , which can ensure that patch_noobj does not affect the detection of other types of targets while attacking specific types of targets.
[0053] Yolov3 is a one-stage detection algorithm, which reconstr...
PUM
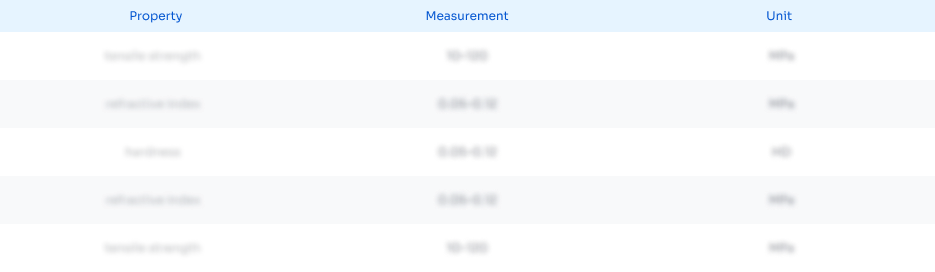
Abstract
Description
Claims
Application Information
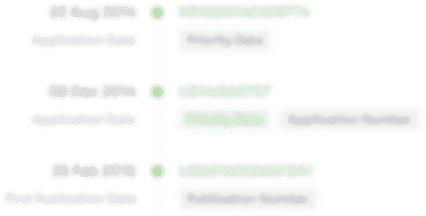
- R&D Engineer
- R&D Manager
- IP Professional
- Industry Leading Data Capabilities
- Powerful AI technology
- Patent DNA Extraction
Browse by: Latest US Patents, China's latest patents, Technical Efficacy Thesaurus, Application Domain, Technology Topic, Popular Technical Reports.
© 2024 PatSnap. All rights reserved.Legal|Privacy policy|Modern Slavery Act Transparency Statement|Sitemap|About US| Contact US: help@patsnap.com