Power load prediction method based on optimal selection of typical daily load curve
A technology of power load and forecasting method, which is applied in the field of power load forecasting based on the optimal selection of typical daily load curves, which can solve the problems of reducing the accuracy of subsequent power load forecasting, the lack of accuracy in the selection of typical daily loads, and the lack of typicality without considering the compactness of sample points In order to achieve the effect of optimizing the clustering effect, improving the clustering accuracy and reducing the operation cost
- Summary
- Abstract
- Description
- Claims
- Application Information
AI Technical Summary
Problems solved by technology
Method used
Image
Examples
Embodiment
[0063] Such as figure 1As shown, a power load forecasting method based on optimal selection of typical daily load curves includes the following steps:
[0064] S1. Acquiring load raw data;
[0065] S2. Preprocessing the original load data to obtain characteristic indicators, specifically performing standardization processing and distortion data screening processing on the original load data in sequence;
[0066] S3. Using the improved PFCM algorithm combined with the fuzzy linear discriminant method (FLDA) to perform cluster analysis on the preprocessed load raw data and determine the cluster center matrix;
[0067] S4. Determine the base date. According to the Pearson correlation coefficient method, calculate the correlation between the base date of each month and each cluster center, and determine the category to which each month belongs. The base date is specifically the monthly average load day;
[0068] S5. Taking the sample point with the highest degree of membership i...
PUM
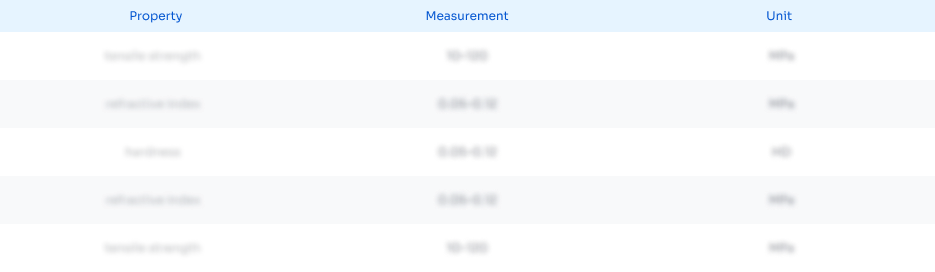
Abstract
Description
Claims
Application Information
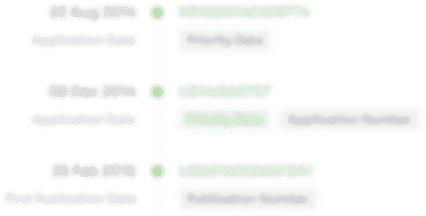
- R&D Engineer
- R&D Manager
- IP Professional
- Industry Leading Data Capabilities
- Powerful AI technology
- Patent DNA Extraction
Browse by: Latest US Patents, China's latest patents, Technical Efficacy Thesaurus, Application Domain, Technology Topic, Popular Technical Reports.
© 2024 PatSnap. All rights reserved.Legal|Privacy policy|Modern Slavery Act Transparency Statement|Sitemap|About US| Contact US: help@patsnap.com