Industrial raw material consumption prediction method based on multi-task time sequence learning
A prediction method and consumption technology, applied in neural learning methods, predictions, biological neural network models, etc., can solve problems such as unsatisfactory raw material consumption, high precision, low cost requirements, unsatisfactory prediction accuracy, and ignoring correlation. Achieve the effect of improving model performance, improving interpretation ability, and enriching constraints
- Summary
- Abstract
- Description
- Claims
- Application Information
AI Technical Summary
Problems solved by technology
Method used
Image
Examples
Embodiment Construction
[0065] The accompanying drawings are for illustrative purposes only and cannot be construed as limiting the patent;
[0066] In order to better illustrate this embodiment, some parts in the drawings will be omitted, enlarged or reduced, and do not represent the size of the actual product;
[0067] For those skilled in the art, it is understandable that some well-known structures and descriptions thereof may be omitted in the drawings.
[0068] The technical solutions of the present invention will be further described below in conjunction with the accompanying drawings and embodiments.
[0069] Such as figure 1 As shown, this patent provides a method for predicting industrial raw material consumption based on multi-task sequential learning, which includes the following steps:
[0070] (1) Formal definition of tasks
[0071] For a given raw material consumption single time series set γ={Y 1 ,Y 2 ,...,Y w}, where Y j (j∈{1,2,...,w}) represents the historical consumption ti...
PUM
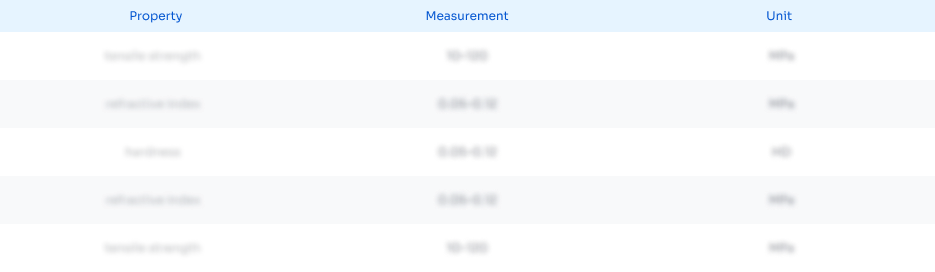
Abstract
Description
Claims
Application Information
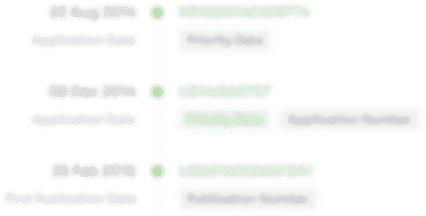
- R&D
- Intellectual Property
- Life Sciences
- Materials
- Tech Scout
- Unparalleled Data Quality
- Higher Quality Content
- 60% Fewer Hallucinations
Browse by: Latest US Patents, China's latest patents, Technical Efficacy Thesaurus, Application Domain, Technology Topic, Popular Technical Reports.
© 2025 PatSnap. All rights reserved.Legal|Privacy policy|Modern Slavery Act Transparency Statement|Sitemap|About US| Contact US: help@patsnap.com