Three-dimensional point cloud semantic segmentation method based on multi-neighborhood features of hybrid model
A technology of neighborhood features and semantic segmentation, applied in neural learning methods, biological neural network models, character and pattern recognition, etc., can solve problems such as poor robustness, and achieve autonomous high robustness 3D point cloud semantic segmentation prediction model Effect
- Summary
- Abstract
- Description
- Claims
- Application Information
AI Technical Summary
Problems solved by technology
Method used
Image
Examples
Embodiment 1
[0055] Such as figure 1 As shown, in one embodiment of the present invention, a kind of 3D point cloud semantic segmentation method based on mixed model multi-neighborhood feature, comprises the following steps:
[0056] S1. Collect point cloud data in three-dimensional space through laser radar, and extract features from the point cloud data to obtain global features of the point cloud, single point features and intermediate features of the network layer;
[0057] S2. Clustering the point cloud data through a clustering algorithm, saving the point indexes in each cluster point subset, and obtaining the intermediate feature cluster subset of the network layer through index classification;
[0058] S3. Perform feature mapping on the intermediate feature cluster subsets of the network layer to obtain high-dimensional multi-neighborhood features corresponding to the intermediate feature cluster subsets of each network layer;
[0059] S4. Splicing and merging point cloud global f...
PUM
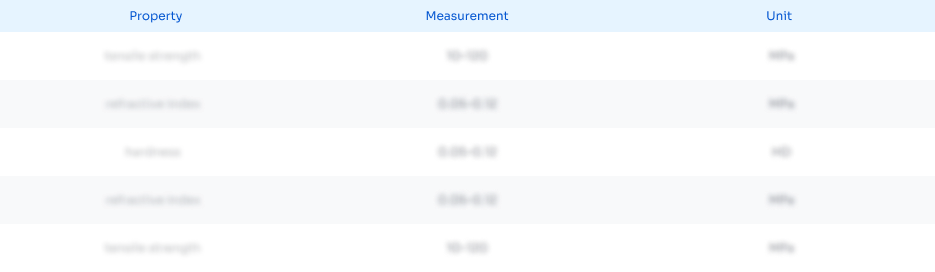
Abstract
Description
Claims
Application Information
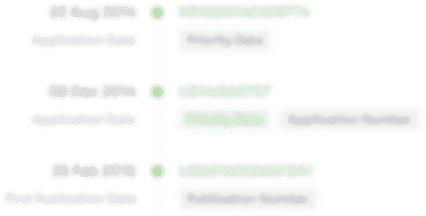
- R&D Engineer
- R&D Manager
- IP Professional
- Industry Leading Data Capabilities
- Powerful AI technology
- Patent DNA Extraction
Browse by: Latest US Patents, China's latest patents, Technical Efficacy Thesaurus, Application Domain, Technology Topic, Popular Technical Reports.
© 2024 PatSnap. All rights reserved.Legal|Privacy policy|Modern Slavery Act Transparency Statement|Sitemap|About US| Contact US: help@patsnap.com