GDP prediction method based on N-BEATS
A forecasting method and preprocessing technology, applied in forecasting, neural learning methods, biological neural network models, etc., can solve the problems of inability to capture nonlinear relationships, forecast errors, and amplify short-term trends, so as to shorten training time and forecast efficiency. The effect of optimizing and reducing the amount of data
- Summary
- Abstract
- Description
- Claims
- Application Information
AI Technical Summary
Problems solved by technology
Method used
Image
Examples
Embodiment Construction
[0031] N-Beats is based on a deep learning algorithm based on trend and seasonal statistical models, and the rear and forward residual links in the model architecture and the very deep all-connection layer stack. The key principle of frame design is:
[0032] First, the infrastructure should be simple and universal, but it is also necessary to explore potential information in depth.
[0033] Second, the architecture should not depend on the feature project or zoom of the input data specific to the time series.
[0034] Third, the architecture should be expandable and can be explained. These principles have reflected in the N-Beats architecture.
[0035] The minimum stack unit in the n-beats model is block, and one block has four full-connection layer stacks. Such as Figure 4 As shown, N-Beats is characterized by using a double residual stack design, and the back and forward two tasks are used to apply the residual design. After the data is input, there is a backward and forward tw...
PUM
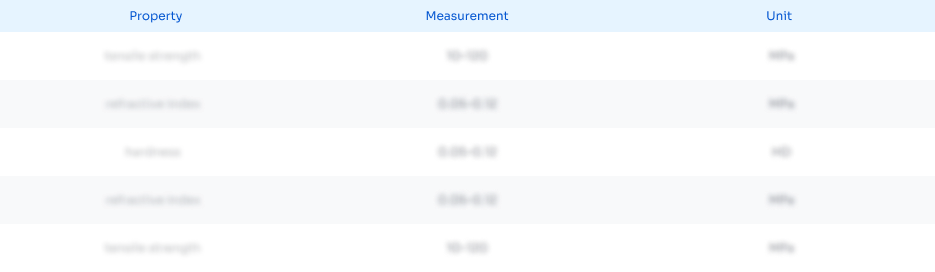
Abstract
Description
Claims
Application Information
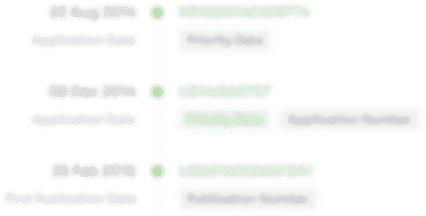
- R&D
- Intellectual Property
- Life Sciences
- Materials
- Tech Scout
- Unparalleled Data Quality
- Higher Quality Content
- 60% Fewer Hallucinations
Browse by: Latest US Patents, China's latest patents, Technical Efficacy Thesaurus, Application Domain, Technology Topic, Popular Technical Reports.
© 2025 PatSnap. All rights reserved.Legal|Privacy policy|Modern Slavery Act Transparency Statement|Sitemap|About US| Contact US: help@patsnap.com