A small sample behavior recognition method and system based on multi-dimensional prototype reconstruction and reinforcement learning
A technology of enhanced learning and recognition methods, applied in the field of computer vision, can solve the problems of weak identification, significant deviation of timing information distribution, and inability to obtain general prototypes, etc., to reduce data deviation, improve discrimination ability, and improve classification accuracy degree of effect
- Summary
- Abstract
- Description
- Claims
- Application Information
AI Technical Summary
Problems solved by technology
Method used
Image
Examples
Embodiment 1
[0051] see figure 1 and 2 As shown, this embodiment provides a small sample behavior recognition method based on multi-dimensional prototype reconstruction and reinforcement learning, including the following steps:
[0052] S10: Calculate the support set time series feature and the query set time series feature based on the obtained support set sample and the query set sample, and use the support set time series feature and the query set time series feature to calculate to obtain the support set video descriptor and the video descriptor of the query set;
[0053] S20: Solve the original time series attention based on the support set time series features, apply a random shuffling and rearrangement operation on the support set time series features, obtain supplementary features of the support set time series features, and calculate the scrambled time series attention of the supplementary features force, perform an inverse operation on the disrupted time sequence attention to ob...
Embodiment 2
[0085] The following describes a small-sample behavior recognition system based on multi-dimensional prototype reconstruction and reinforcement learning disclosed in Embodiment 2 of the present invention. The small-sample behavior recognition system based on multi-dimensional prototype reconstruction and reinforcement learning described below is the same as the one described above. A few-shot behavior recognition method based on multi-dimensional prototype reconstruction reinforcement learning can be referred to each other.
[0086] see Image 6 As shown, the second embodiment of the present invention discloses a small sample behavior recognition system based on multi-dimensional prototype reconstruction and reinforcement learning, including:
[0087] A video descriptor calculation module 100, the video descriptor calculation module 100 is configured to calculate the support set time series features and the query set time series features based on the obtained support set sampl...
PUM
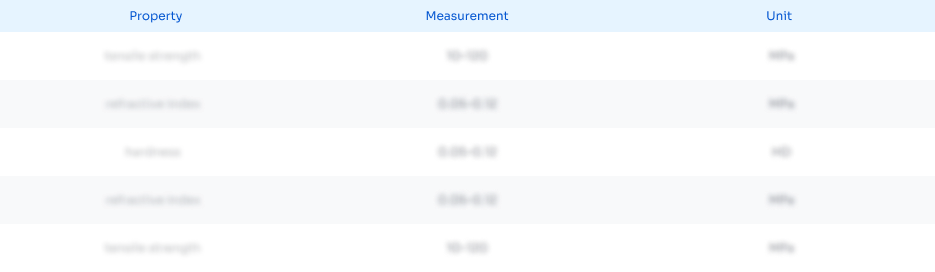
Abstract
Description
Claims
Application Information
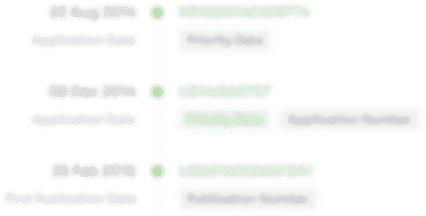
- R&D Engineer
- R&D Manager
- IP Professional
- Industry Leading Data Capabilities
- Powerful AI technology
- Patent DNA Extraction
Browse by: Latest US Patents, China's latest patents, Technical Efficacy Thesaurus, Application Domain, Technology Topic, Popular Technical Reports.
© 2024 PatSnap. All rights reserved.Legal|Privacy policy|Modern Slavery Act Transparency Statement|Sitemap|About US| Contact US: help@patsnap.com