Federal learning-based human activity identification method and system
A human activity and recognition method technology, applied in the field of human activity recognition, can solve the problems of inability to protect user data privacy and insufficient recognition result accuracy, and achieve the effect of optimizing model aggregation effect and improving recognition accuracy.
- Summary
- Abstract
- Description
- Claims
- Application Information
AI Technical Summary
Problems solved by technology
Method used
Image
Examples
Embodiment 2
[0044] On the basis of the above-mentioned embodiments, the embodiment of the present invention also provides a human activity recognition method based on federated learning (called P-FEDAVG), including the following steps:
[0045] S201: The server determines the training task and the corresponding activity data features, generates initial model parameters, and sends the initial model parameters to each client;
[0046] S202: Each client performs local training according to the received initial model parameters or new global model parameters in combination with its own local activity data, generates local update parameters, and uploads the local update parameters to the server;
[0047] When training in combination with local activity data and current model parameters, the multi-category cross-entropy loss function categorical_crossentropy is used and softmax is introduced as a classifier to identify activities.
[0048] As an implementable mode, each client uses the gradient d...
Embodiment 3
[0065] combine figure 1 As shown, the embodiment of the present invention also provides a human activity recognition system based on federated learning, including: a server and multiple clients; multiple clients establish connections and communicate with the server through Ethernet;
[0066] The server is configured to determine the training tasks and corresponding activity data features, and generate initial model parameters, and send the initial model parameters to each client; and perform weighted average aggregation of the received local update parameters of each client to generate new global model parameters, and send the new global model parameters to each client;
[0067] Each client is configured to perform local training in combination with its own local activity data according to the received initial model parameters or new global model parameters, generate local update parameters, and upload the local update parameters to the server.
[0068] It should be noted tha...
PUM
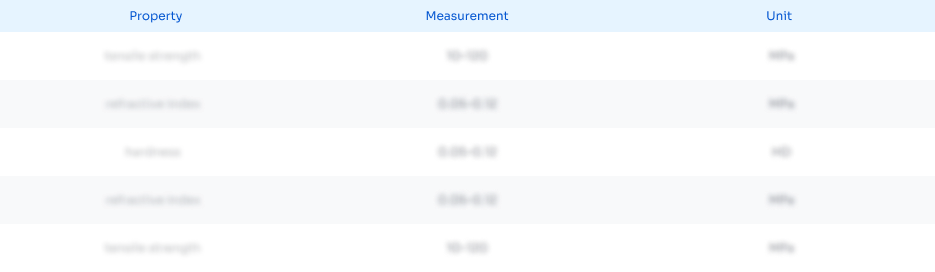
Abstract
Description
Claims
Application Information
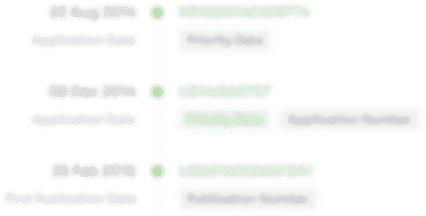
- R&D Engineer
- R&D Manager
- IP Professional
- Industry Leading Data Capabilities
- Powerful AI technology
- Patent DNA Extraction
Browse by: Latest US Patents, China's latest patents, Technical Efficacy Thesaurus, Application Domain, Technology Topic, Popular Technical Reports.
© 2024 PatSnap. All rights reserved.Legal|Privacy policy|Modern Slavery Act Transparency Statement|Sitemap|About US| Contact US: help@patsnap.com