Small sample semi-supervised learning method and device based on pseudo label noise filtering
A semi-supervised learning and noise filtering technology, applied in neural learning methods, character and pattern recognition, instruments, etc., can solve problems affecting the performance of the main model, and achieve the effect of high model optimization efficiency and strong versatility
- Summary
- Abstract
- Description
- Claims
- Application Information
AI Technical Summary
Problems solved by technology
Method used
Image
Examples
Embodiment Construction
[0041] The present invention will be described in detail below in conjunction with the accompanying drawings and specific embodiments. This embodiment is carried out on the premise of the technical solution of the present invention, and detailed implementation and specific operation process are given, but the protection scope of the present invention is not limited to the following embodiments.
[0042] This embodiment provides a small-sample semi-supervised learning method based on pseudo-label noise filtering, which is applied to image classification problems in the field of computer vision. The process is as follows figure 1 As shown, it specifically includes the following steps:
[0043] Step S1. Obtain the initial labeled dataset L s and the initial unlabeled dataset U s , L s ={(x i ,y i )}, U s ={x j}. Among them, these data sets are all derived from the miniimagenet image data set, and a total of 50,000 training images of 100 categories are selected as the init...
PUM
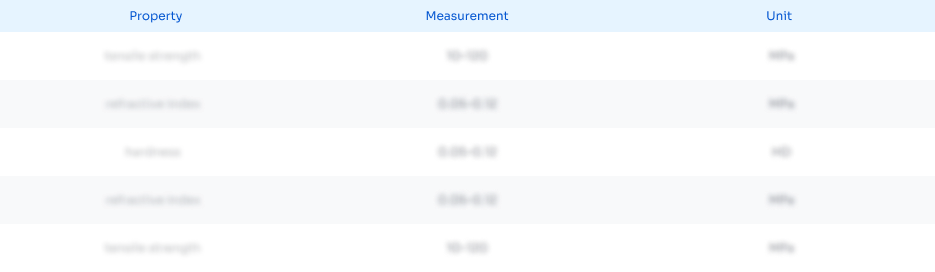
Abstract
Description
Claims
Application Information
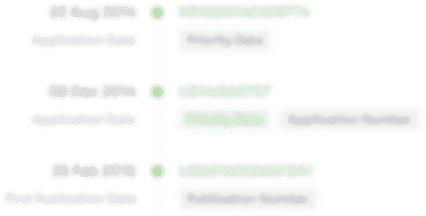
- R&D Engineer
- R&D Manager
- IP Professional
- Industry Leading Data Capabilities
- Powerful AI technology
- Patent DNA Extraction
Browse by: Latest US Patents, China's latest patents, Technical Efficacy Thesaurus, Application Domain, Technology Topic, Popular Technical Reports.
© 2024 PatSnap. All rights reserved.Legal|Privacy policy|Modern Slavery Act Transparency Statement|Sitemap|About US| Contact US: help@patsnap.com