Self-supervised attribute controllable image generation method based on deep twin network
A twin network and image generation technology, applied in the field of computer vision, can solve the problems of large model parameters, complex network optimization steps, and insufficient category attribute control effect, so as to improve reliability, improve category attribute control effect and image generation quality , good category attribute to control the effect of image generation effect
- Summary
- Abstract
- Description
- Claims
- Application Information
AI Technical Summary
Problems solved by technology
Method used
Image
Examples
Embodiment Construction
[0084] Step 1: Preprocessing the experimental data;
[0085] The present invention selects the CIFAR-10 data set as the experimental data, which is obtained from the official release channel of the data set. The CIFAR-10 dataset consists of 60,000 true-color RGB images, of which 50,000 are training data and the remaining 10,000 are testing data. The CIFAR-10 dataset contains a total of 10 category attributes, and each category attribute has the same number of images. In addition, each image in the dataset is 3×32×32 in size. In order to apply RGB image data to the training of the deep learning model, convert all image data into Tensor form.
[0086] Step 2: Carry out image transformation operation;
[0087] The experimental image data will be applied to the training of the generative confrontation network and the training of the twin encoder network for decoupling attribute separation learning. For the training of the generative confrontation network, the Tensor format data ...
PUM
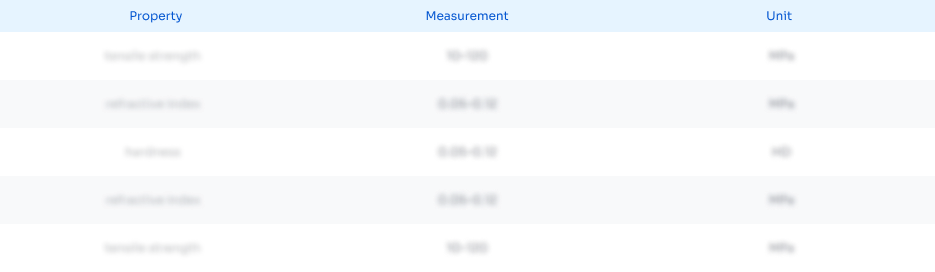
Abstract
Description
Claims
Application Information
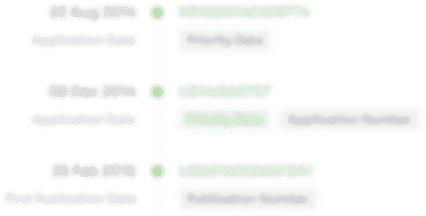
- R&D Engineer
- R&D Manager
- IP Professional
- Industry Leading Data Capabilities
- Powerful AI technology
- Patent DNA Extraction
Browse by: Latest US Patents, China's latest patents, Technical Efficacy Thesaurus, Application Domain, Technology Topic.
© 2024 PatSnap. All rights reserved.Legal|Privacy policy|Modern Slavery Act Transparency Statement|Sitemap