Strong generalization eye fundus image segmentation method based on semi-supervised learning
A semi-supervised learning and fundus image technology, applied in the field of computer vision, can solve the problems of lack of generalization ability, expensive, laborious, etc., and achieve the effect of improving recognition effect and solving labeling problems
- Summary
- Abstract
- Description
- Claims
- Application Information
AI Technical Summary
Problems solved by technology
Method used
Image
Examples
Embodiment Construction
[0035] In order to make the technical means, creative features, goals and effects realized by the present invention easy to understand, the following describes the semi-supervised learning-based strong generalization fundus image segmentation method of the present invention in conjunction with the embodiments and the accompanying drawings.
[0036] In the description of the present invention, the terms "first" and "second" are used for descriptive purposes only, and cannot be understood as indicating or implying relative importance.
[0037]
[0038] In this embodiment, the strong generalization fundus image segmentation method based on semi-supervised learning is run through a computer, and the computer needs a graphics card for GPU acceleration to complete the training process of the model, and the trained fundus image segmentation model and fundus The image segmentation process and results are stored in the computer.
[0039] In this embodiment, the data sets used include...
PUM
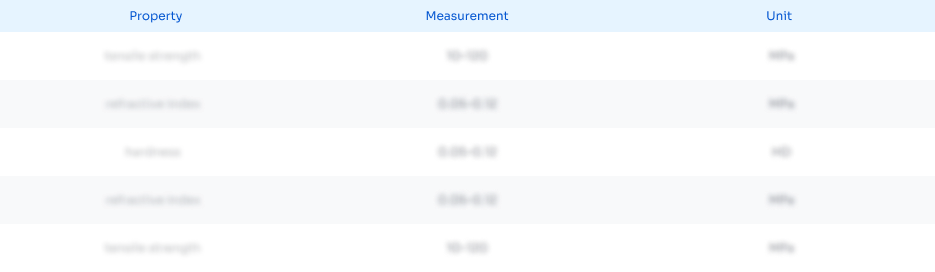
Abstract
Description
Claims
Application Information
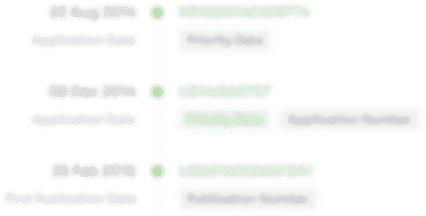
- R&D Engineer
- R&D Manager
- IP Professional
- Industry Leading Data Capabilities
- Powerful AI technology
- Patent DNA Extraction
Browse by: Latest US Patents, China's latest patents, Technical Efficacy Thesaurus, Application Domain, Technology Topic, Popular Technical Reports.
© 2024 PatSnap. All rights reserved.Legal|Privacy policy|Modern Slavery Act Transparency Statement|Sitemap|About US| Contact US: help@patsnap.com