Lithium ion battery thermal runaway early warning method based on deep learning
A lithium-ion battery and deep learning technology, applied in the field of thermal runaway warning of lithium-ion batteries, can solve problems such as personal and property losses, battery short circuit, thermal runaway, etc., and achieve the goal of promoting intelligent progress, accelerating application, and improving speed and accuracy Effect
- Summary
- Abstract
- Description
- Claims
- Application Information
AI Technical Summary
Problems solved by technology
Method used
Image
Examples
Example Embodiment
[0035]为了使本发明的目的、技术方案和优点更加清楚,下面结合具体实施例对本发明进行详细描述。在此,还需要说明的是,为了避免因不必要的细节而模糊了本发明,在具体实施例中仅仅示出了与本发明的方案密切相关的结构和 / 或处理步骤,而省略了与本发明关系不大的其他细节。
[0036]另外,还需要说明的是,术语“包括”、“包含”或者其任何其他变体意在涵盖非排他性的包含,从而使得包括一系列要素的过程、方法、物品或者设备不仅包括那些要素,而且还包括没有明确列出的其他要素,或者是还包括为这种过程、方法、物品或者设备所固有的要素。
[0037]参阅图1所示,本发明提供的一种深度学习的锂离子电池热失控预警方法,包括以下步骤:
[0038]S1.获取锂电池的原始数据集:采集锂电池的电池数据,并将电池数据分为外部环境数据、文本数据及电池老化数据;所述外部环境数据为时间序列数据,所述文本数据为维修记录,所述电池老化数据包括电池电压、电流、温度及电池容量;
[0039]S2.获取外部环境数据和文本数据的融合特征:外部环境数据E,采用 min-max归一化将数据缩放到0和1之间,利用LSTM提取外部环境信息的潜在特征,环境特征记为E∈Rk;将维修记录利用one-hot编码方式转换成矩阵形式 [M1,M2,...,Mn],Mn表示第n个单词的one-hot编码向量,同样利用LSTM提取文本信息的潜在特征,文本特征记为M∈Rk,将外部环境数据E及文本数据M 拼接,记为外部特征O=[E,M]∈R2k,将外部特征O作为全连接网络的输入,非线性变换得到外部环境和文本的融合特征O′∈Rk;
[0040]S3.提取和选择相关健康指标:利用式1所示的相关系数矩阵,计算电压、电流和温度相关特征与电池容量的相关系数ρ,通过设计阈值threshold,如果ρ>threshold则为关键特征,ρ<threshold则筛选掉;
[0041]
[0042]其中,X是特征值,Y是目标值,μX和μY是平均值
[0043]S4.获取关键特征的时间特征向量:将每个关键特征输入门控循环单元 GRU,再经过全连接网络得到关键特征的时间特征向量,记为H∈Rk;
[0044]S5.获取图卷积网络的输入:采用卷积神经网络CNN提取关键特征的局部特征,得到特征矩阵X∈Rn×k,n为关键特征个数,k为时间特征向量维度;计算关键特征的特征向量间的欧氏距离,利用...
PUM
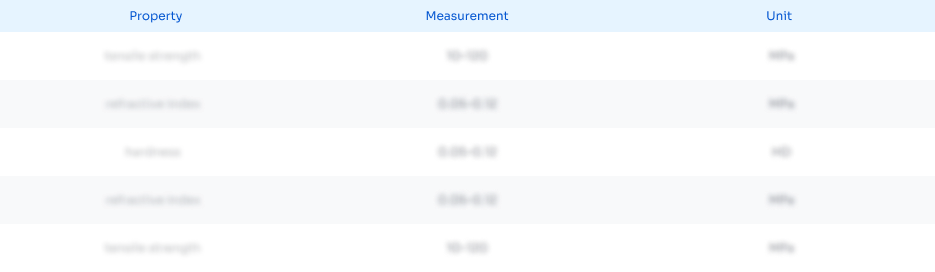
Abstract
Description
Claims
Application Information
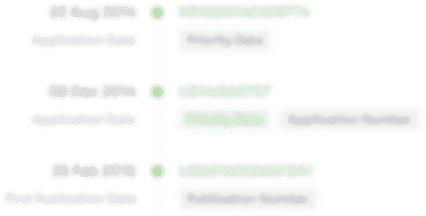
- R&D Engineer
- R&D Manager
- IP Professional
- Industry Leading Data Capabilities
- Powerful AI technology
- Patent DNA Extraction
Browse by: Latest US Patents, China's latest patents, Technical Efficacy Thesaurus, Application Domain, Technology Topic, Popular Technical Reports.
© 2024 PatSnap. All rights reserved.Legal|Privacy policy|Modern Slavery Act Transparency Statement|Sitemap|About US| Contact US: help@patsnap.com