Machine Learning System
- Summary
- Abstract
- Description
- Claims
- Application Information
AI Technical Summary
Benefits of technology
Problems solved by technology
Method used
Image
Examples
Embodiment Construction
[0066]Referring now to FIG. 1, which illustrates a prior art Active Learning system for the classification of documents. Whilst the present invention is described with reference to the classification of documents it will be clear to those skilled in the art that the system described herein is equally applicable to othe machine learning applications where elements of a data set must be reliably classified according to a characteristic of that element.
[0067]Corpus 100 is a data set consisting of a plurality of text documents such as web pages. In practice each document is represented by a vector d=[ω1, . . . ωN] which is an element of the high-dimensional vector-space consisting of all terms. In this representation ωi is non-zero for document d only if the document contains term ti. The numerical value of wi can be set in a variety of ways, ranging from simply setting it to 1, regardless of the frequency of ti in d, through to the use of more sophisticated weighting schemes such as tf...
PUM
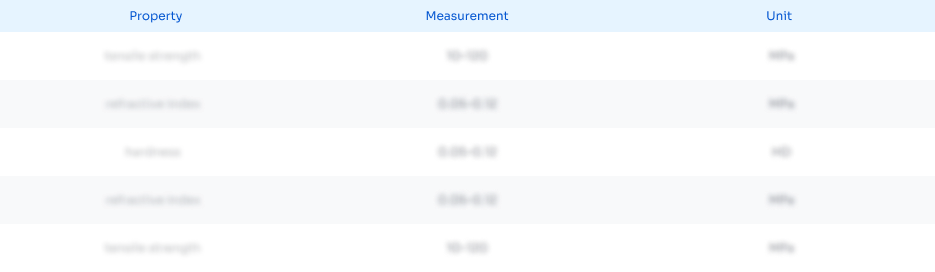
Abstract
Description
Claims
Application Information
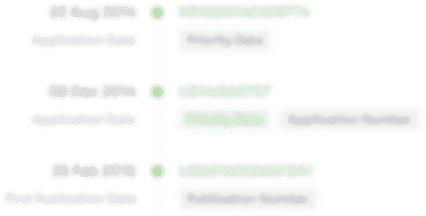
- R&D Engineer
- R&D Manager
- IP Professional
- Industry Leading Data Capabilities
- Powerful AI technology
- Patent DNA Extraction
Browse by: Latest US Patents, China's latest patents, Technical Efficacy Thesaurus, Application Domain, Technology Topic, Popular Technical Reports.
© 2024 PatSnap. All rights reserved.Legal|Privacy policy|Modern Slavery Act Transparency Statement|Sitemap|About US| Contact US: help@patsnap.com